Data Sharing in Research: Implications and Opportunities
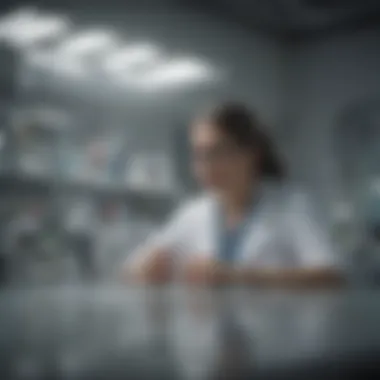
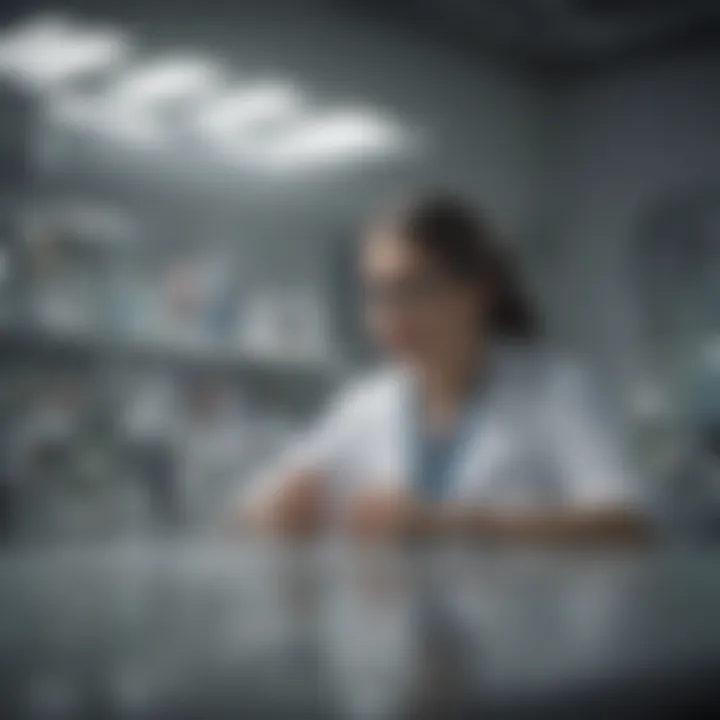
Intro
In the rapidly evolving landscape of research, data sharing has emerged as a crucial phenomenon, impacting various scientific fields. This article delves into the complexities surrounding data sharing for research purposes, highlighting its implications and opportunities. With a careful look at the potential benefits and the ethical challenges, it aims to foster a deeper understanding of how transparent data sharing fosters collaboration and innovation.
A key focus is placed on understanding the frameworks that govern data sharing practices. Institutions, researchers, and the scientific community play integral roles in shaping these practices. By examining current policies, technologies, and relevant case studies, this article offers valuable insights into enhancing research outcomes through shared data. Ultimately, it seeks to inform stakeholders about their responsibilities and opportunities in promoting effective data-sharing practices.
Methodology
Overview of Research Methods Used
The research conducted for this article draws upon a mixed-methods approach. Qualitative data was gathered through interviews and discussions with professionals engaged in research, while quantitative data emerged from analyzing existing literature and research policies. This approach allows for a comprehensive examination of both personal insights and broader patterns in data-sharing practices.
Data Collection Techniques
Data collection involved the following techniques:
- Interviews: Engaging researchers and educators revealed their perspectives on data sharing.
- Surveys: Gathering responses from institutions provided a quantitative view of existing policies.
- Literature Review: Analyzing academic journals and case studies enriched the understanding of the current landscape of data-sharing practices.
These methods contribute to informing stakeholders about effective data-sharing strategies and their implications.
Implications of Data Sharing
The implications of data sharing in research extend across various dimensions. Benefits include:
- Enhanced Collaboration: Sharing data allows diverse teams to work together, leading to innovative outcomes.
- Increased Transparency: Open data promotes trust and accountability among researchers and institutions.
- Accelerated Innovation: Access to shared data can lead to new insights and accelerate discoveries.
However, challenges must be acknowledged, such as:
- Ethical Concerns: Protecting sensitive information is vital to maintain participant privacy.
- Data Quality Issues: Ensuring accuracy and reliability in shared data is critical.
Future Directions
Upcoming Trends in Research
The future of data sharing in research is promising, with several trends beginning to take shape:
- Cloud-Based Platforms: Researchers increasingly utilize cloud technology for data sharing, enhancing accessibility.
- Interdisciplinary Collaboration: Different fields are increasingly merging, leading to more comprehensive studies.
Areas Requiring Further Investigation
There are still areas that need more exploration:
- Ethical Frameworks: Developing robust guidelines to address data privacy will be crucial.
- Standardization of Practices: Creating uniform data-sharing protocols can enhance overall efficiency.
This article serves as a comprehensive guide for stakeholders, illustrating the significance of data sharing in advancing research while highlighting the responsibilities surrounding it.
Overall, understanding the implications and opportunities of data shared for research fosters an environment where innovation and collaboration thrive.
Preamble to Data Sharing in Research
Data sharing in research is a fundamental aspect that promotes transparency, collaboration, and innovation within the scientific community. As research methodologies evolve, the need for accessible data becomes more paramount. This section explores the significance of data sharing, emphasizing its implications and opportunities that arise from effective practices.
Defining Data Sharing
Data sharing refers to the practice of making data available for use by others within the research community. This can include sharing datasets, findings, and research tools across various platforms. By defining data sharing, we address a crucial part of research integrity, where the availability of data allows for replication of results and further exploration of scientific inquiries. In essence, data sharing fosters a culture of openness and accountability, contrasting with traditional models where results are often held behind institutional or individual barriers.
Examples of data sharing can be seen in repositories such as the Open Science Framework, where researchers upload their datasets to enable others to access and utilize them. This openness enhances the potential for collaborative work, thereby driving innovation across various disciplines.
Historical Context of Data Sharing
The historical context of data sharing reveals a gradual shift in how researchers perceive and engage with data. Initially, data was seen as proprietary, guarded closely by researchers and institutions. However, as the internet and technology advanced, the paradigms began to shift.
In the late 20th century, initiatives arose encouraging data sharing, spearheaded by organizations advocating for open science. This shift was largely influenced by the recognition that unshared data limits scientific progress and collaboration. Furthermore, notable moments such as the Human Genome Project illustrated the benefits of data sharing, resulting in rapid advancements in genetics that would not have been possible without a shared approach.
Today, data sharing has become increasingly common, supported by funding agencies and institutions that require transparency in research practices. As a result, both historical context and contemporary practices highlight the ongoing evolution toward a more inclusive approach in research.
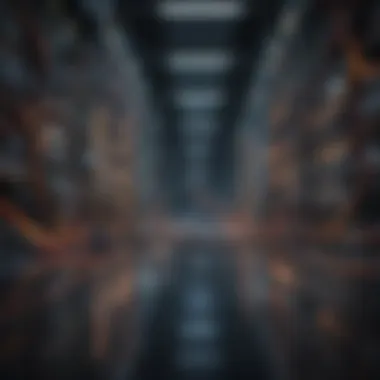
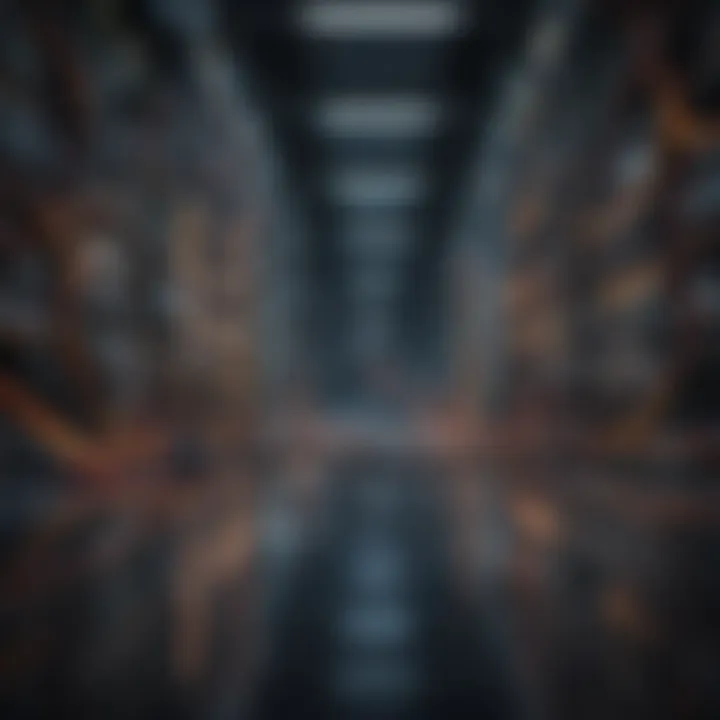
The Importance of Data Sharing
Data sharing is a fundamental aspect of the modern research landscape. As academic and professional environments increasingly prioritize collaboration and transparency, the role of data sharing holds significant weight. Clear communication of data practices fosters trust among researchers. This trust can stimulate broader partnerships that enhance research outcomes. By sharing data, scholars can build on existing work, leading to multifaceted contributions across disciplines.
Through shared data, researchers can also avoid duplication of efforts. Instead of reinventing the wheel, projects can proceed with an enhanced understanding of prior findings. That connection minimizes wasted resources, allowing teams to channel their energy toward new inquiries.
Enhancing Research Collaboration
Collaboration among researchers brings diverse perspectives, expertise, and resources together. When researchers share data, it facilitates teamwork across various fields. Collaboration helps to break down silos within disciplines, prompting interdisciplinary projects. These projects often yield innovative results that benefit the wider scientific community.
Moreover, sharing data encourages partnerships that can include universities, industries, government agencies, and non-profits. This expanded network creates a rich exchange of ideas, elevating the quality of research produced. Shared data can also become a common ground for verification, fostering a sense of community around scientific inquiry.
"Data sharing becomes not just a means of collaboration but a catalyst for joint innovation, often resulting in outcomes unattainable through isolated efforts."
Through enhanced collaboration, researchers can utilize different methodologies and tools. This synergy can enhance the complexity and richness of the research. In turn, this may lead to groundbreaking discoveries that can have real-world impacts. The collaborative nature of shared data also plays a role in educating the next generation of scholars. Students involved in these initiatives benefit from firsthand experience in diverse teams and best practices of data use.
Accelerating Scientific Discovery
Scientific discovery hinges on the ability to access and utilize accumulated knowledge. Data sharing expedites this process by making findings readily available. When researchers share datasets, it allows others to test hypotheses and replicate studies more efficiently. This element is crucial for advancing scientific progress, as verification of results is a cornerstone of reliable research.
By having access to a broader array of data, scientists can see patterns and correlations that may not have been clear in isolated studies. This accessibility enhances the potential for leading-edge discoveries and innovative solutions in various fields, including medicine, technology, and environmental science.
Additionally, shared data can drive the development of machine learning and AI applications. With vast datasets available, researchers can train algorithms that identify trends and insights faster than human analysis alone. This accelerates the research process and opens doors for breakthroughs that may not have been possible with smaller datasets.
Models of Data Sharing
The concept of data sharing has increasingly become a necessary practice within various domains of research. This section addresses two primary models of data sharing: open data initiatives and controlled access data repositories. Understanding these models is crucial as they represent distinct approaches with different implications for accessibility, privacy, and the impact on research integrity.
Open Data Initiatives
Open data initiatives are designed to promote free access to data generated from various research activities. The premise is founded on the belief that sharing data openly leads to enhanced collaboration, transparency, and innovation. By making research data publicly available, researchers can enable others to validate findings, build upon existing work, and sometimes even discover new insights.
The benefits of open data initiatives include:
- Increased Visibility: Open data can enhance the profile of researchers as their work reaches a broader audience.
- Encouragement of Collaboration: It fosters interdisciplinary collaboration, allowing different fields to intersect.
- Replication Opportunities: Other researchers can replicate studies, ensuring the robustness of the original findings and increasing the credibility of research.
However, there are considerations to weigh. Not all data can or should be open. Issues such as data privacy, potential for misuse, or ethical constraints must be navigated carefully. The model works best when there are clear guidelines on what data can be shared. A focus on anonymization procedures is particularly important to protect personal information.
Controlled Access Data Repositories
In contrast, controlled access data repositories provide a more restricted mechanism for sharing data. These repositories allow for data to be accessed only by individuals who meet certain criteria. This model is particularly relevant for sensitive data where privacy and ethical commitments are paramount.
The key features of controlled access data repositories are:
- Enhanced Data Security: Sensitive information remains protected, as access is limited to authorized individuals.
- Compliant with Regulations: Such systems can adhere better to legal and institutional guidelines surrounding data use, particularly in fields like healthcare or social sciences.
- Contextual Access: Researchers can access data relevant to specific projects without compromising the integrity of sensitive cases.
Challenges associated with controlled access include:
- Barrier to Access: Potentially limiting the ability of researchers to utilize data, which could slow the pace of scientific discovery.
- Management Complexity: Maintaining such a repository requires robust systems and protocols to manage access permissions and ensure compliance with privacy standards.
In essence, both models of data sharing facilitate different aspects of research and innovation. By evaluating the context and the type of data being shared, researchers can choose the appropriate model that balances access with ethical considerations. This understanding is vital as the academic world continues to seek effective ways to utilize shared data for maximizing research impacts.
Ethical Considerations in Data Sharing
Understanding the implications of data sharing goes beyond just technical and structural aspects. Ethical considerations play a significant role in shaping how data is collected, stored, and utilized in research. Establishing clear ethical guidelines ensures that researchers respect the rights and privacy of data subjects, which is essential for maintaining trust in the research process. The importance of ethics in data sharing cannot be overstated as it guides practitioners in navigating the complex landscape of shared data. By emphasizing ethical principles, researchers can foster a culture of responsibility and transparency that benefits the entire scientific community.
Informed Consent and Data Privacy
Informed consent is fundamental in the realm of data sharing. Individuals providing their data must understand how their information will be used. This transparency is necessary to build trust between researchers and participants. Clear communication can prevent misunderstandings and promote ethical practices within research.
If individuals are not adequately informed, they cannot make educated decisions regarding their participation. Researchers should clearly outline the purpose of the data collection, the types of data being collected, and potential risks or benefits involved.
The principles of data privacy come into play when considering how personal information is handled. The use of anonymization techniques is one way to protect individual identities while still utilizing data sets for research. Moreover, regulatory frameworks like the General Data Protection Regulation (GDPR) underscore the necessity of strict privacy measures. Adhering to these regulations is not just a legal formality; it serves as a standard that promotes ethical research practices.
"Ethical research practices build trust among participants and enhance the credibility of scientific inquiry."
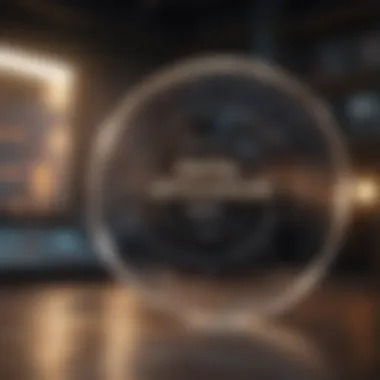
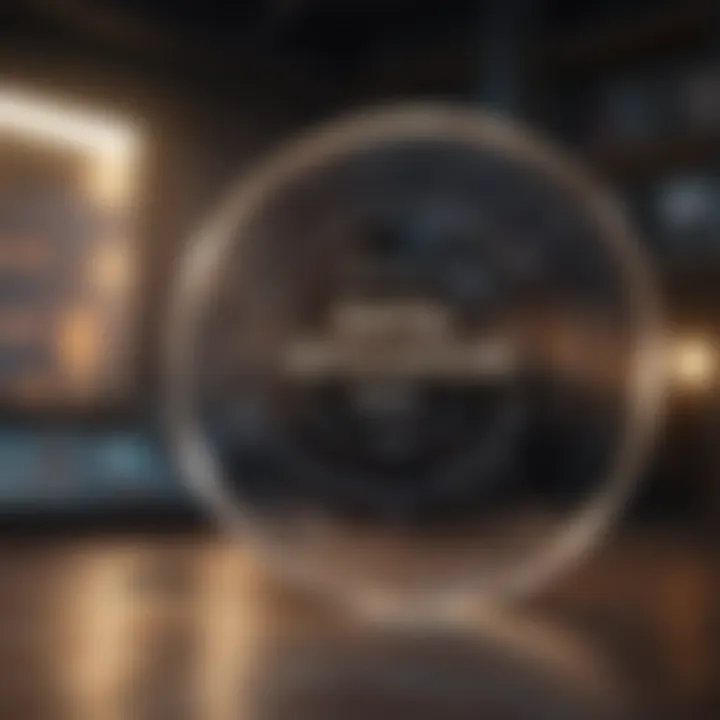
Handling Sensitive Data
Handling sensitive data creates additional ethical challenges. Researchers must identify what constitutes sensitive data, which may include health records, financial information, or details that could lead to discrimination. Special care should be taken to mitigate risks associated with the disclosure of such information. Establishing clear protocols for data handling, storage, and sharing is essential.
The use of controlled access data repositories allows researchers to regulate who can view or use sensitive data. Access can be limited only to individuals who have a legitimate need to know or who are involved in specific research projects. This approach is vital in protecting participants from potential harm due to data misuse.
Furthermore, ongoing training for researchers in ethical data handling practices is crucial. Awareness and education can significantly help in preventing unintentional ethical breaches. Institutions should invest in training programs to ensure all stakeholders understand the importance of ethical considerations in the data-sharing context.
In summary, ethical considerations are central to data sharing in research. By prioritizing informed consent and diligently handling sensitive data, researchers can contribute to a responsible and trustworthy research landscape.
Keywords such as "data sharing ethics," "informed consent," and "data privacy" are essential for search optimization, ensuring that this crucial information is easily accessible for those seeking knowledge in the field.
Technological Advances in Data Sharing
Technological advances play a crucial role in enhancing data sharing practices within research. These innovations not only streamline processes but also address many of the challenges currently faced in research data sharing. With advancements in technology, the flow of information has become more efficient and accessible. As researchers increasingly rely on digital systems, it is important to examine the specific technologies that enable effective data sharing.
Cloud-based Platforms
Cloud-based platforms represent a significant leap forward in the way researchers store, access, and share data. These platforms allow for scalable storage solutions, which accommodate the vast amounts of data generated by contemporary research. Solutions such as Google Drive, Dropbox, and Amazon Web Services provide users with flexibility. Researchers can access their data from virtually anywhere, enhancing collaboration across locations and disciplines.
Furthermore, cloud technologies enable real-time data sharing among multiple users. This fosters collaboration and accelerates the research process. Feedback can be immediate, and researchers can iterate on projects more quickly. Importantly, cloud platforms often include security features that protect sensitive data, ensuring compliance with research regulations.
Blockchain Technology in Data Management
Blockchain technology has the potential to revolutionize data management in research settings. By providing a decentralized method for storing data, blockchain enhances transparency and trust. Each transaction is recorded in a secure and immutable ledger, allowing participants to access a verified history of data changes. This can be particularly beneficial for data integrity, crucial in research contexts where the accuracy of information is non-negotiable.
Moreover, blockchain can facilitate better data sharing agreements among institutions. Smart contracts can automate and enforce terms related to data access and use. This not only simplifies compliance but also builds a foundation of accountability between researchers.
As research continues to evolve, embracing technological advances is essential. These innovations carry the potential to address existing challenges and unlock new opportunities for collaboration and discovery.
Data Sharing Policies
Data sharing policies play a critical role in shaping the landscape of research collaboration and innovation. These policies ensure clear guidelines for sharing data while balancing the need for transparency with ethical obligations and privacy concerns. Effective data sharing policies foster trust among researchers, institutions, and stakeholders, enabling a robust research ecosystem.
Institutional Guidelines and Frameworks
Institutional guidelines provide a structured approach to data sharing within research. These frameworks are essential for outlining the responsibilities of researchers concerning data usage and management. They also set the rules for data access, ensuring that all parties comply with legal and ethical standards.
- Standardization of Practices: A well-defined framework leads to standardized data practices, which enhances the reproducibility of research. This is especially important in fields such as medicine and social sciences, where data integrity is paramount.
- Risk Management: Institutional guidelines also help to mitigate risks associated with data breaches. By establishing protocols for data handling and storage, institutions can protect sensitive information, thereby safeguarding the privacy of individuals involved in research.
- Accountability: Guidelines enhance accountability among researchers. By outlining what is expected in terms of data sharing, institutions can hold researchers responsible for adhering to these standards, which can protect institutions from potential legal issues.
- Training and Support: Institutions often provide training for researchers on these guidelines. This ensures that researchers are equipped to share data responsibly and comply with legal obligations.
In summary, institutional guidelines create a cohesive framework for data sharing and promote ethical practices while protecting both researcher and participant interests.
Funding Agency Requirements
Funding agencies have significant influence on data sharing practices by setting requirements that researchers must adhere to when applying for grants. These requirements encourage open science and enhance the visibility and usability of research data.
- Mandatory Data Management Plans: Many funding agencies require researchers to submit data management plans that detail how data will be collected, shared, and archived. These plans ensure that researchers carefully consider how data will be disposed of after the project ends, facilitating long-term preservation.
- Transparency and Accessibility: Funding agencies often push for more transparent data practices. They may mandate that researchers make their data publicly available or submit it to central repositories, thereby enhancing accessibility for future research.
- Promotion of Collaborative Research: By emphasizing the importance of data sharing, funding agencies help to foster collaborative efforts across disciplines. This can lead to interdisciplinary studies that tackle complex research questions.
- Evaluation Criteria: Funding agencies may assess data sharing practices as part of the grant evaluation process. Projects that demonstrate a strong commitment to data sharing may receive priority funding, incentivizing researchers to adopt effective data-sharing practices.
Case Studies of Successful Data Sharing
Case studies serve as vital illustrations of how data sharing can foster innovation and collaboration in research. They provide real-world examples that can guide future initiatives, showing both the benefits and challenges that accompany data sharing. Through these case studies, researchers can identify effective strategies and adapt successful frameworks that enhance their projects.
One notable advantage of these studies is their ability to showcase successful outcomes that can inspire change in similar contexts. They highlight how various fields can leverage shared data to advance knowledge and solve complex issues.
Moreover, case studies often reveal the importance of policies, cultural dynamics, and technological infrastructures necessary for successful data sharing. Insights gained from these examples can draw attention to the need for clear communication across disciplines, ensuring that the benefits of shared data extend to all stakeholders involved in the research process.
Data sharing allows for enhanced collaboration, which is particularly beneficial in a landscape that is increasingly interdisciplinary.
Collaborative Projects in Medicine
In the medical field, collaborative data-sharing projects are paramount. They not only enhance the efficiency of research but also contribute to more robust findings. For instance, the Global Alliance for Genomics and Health exemplifies how data sharing facilitates international partnerships, allowing researchers to access genomic data from various populations. This collective effort leads to improved understanding of disease patterns and has the potential to accelerate the development of targeted therapies.
Another successful example comes from the COVID-19 pandemic. Researchers quickly shared data about the virus's spread and its implications on health outcomes. Initiatives like the COVID-19 Open Research Dataset enabled scientists globally to collaborate, analyze, and interpret data to implement effective public health responses rapidly. This urgent sharing of information can be credited for accelerating vaccine development, showcasing how timely data sharing can save lives.
Interdisciplinary Research Initiatives
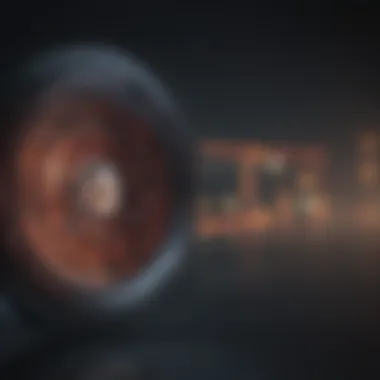
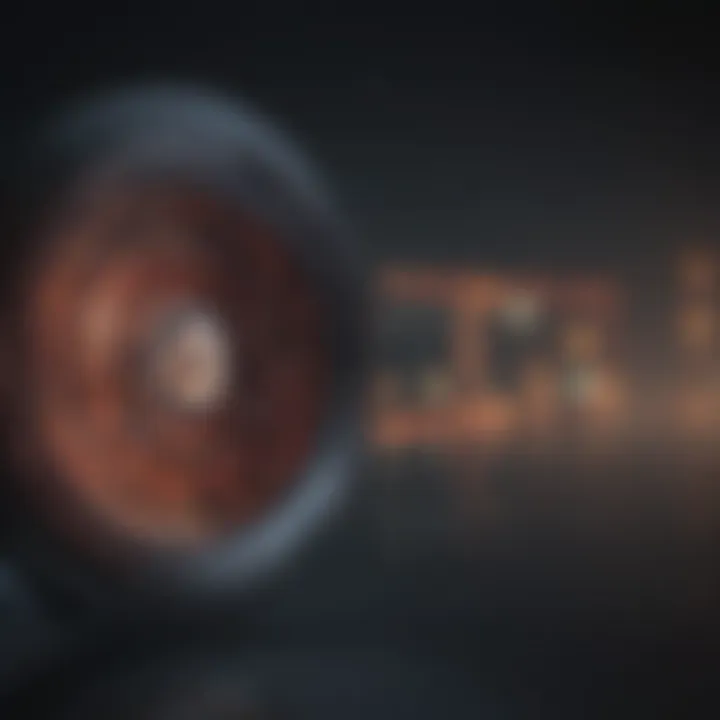
Interdisciplinary research initiatives often thrive on effective data sharing. By blending knowledge from different fields, researchers can address complex problems more holistically. For instance, projects that combine environmental data with health studies have provided valuable insights into how pollution affects public health. The integration of data from social sciences, natural sciences, and health research can create a comprehensive understanding of such challenges.
The National Institutes of Health's Precision Medicine Initiative is another example where interdisciplinary collaboration benefitted from shared data. By combining information from various medical fields, participants can better tailor treatments and interventions for patients, leading to improved health outcomes. The sharing of diverse datasets fosters an environment where innovative solutions can emerge, reflecting the collective intelligence of various disciplines.
Overall, these case studies highlight the transformative potential of data sharing. They present a strong case for its adoption as a central element in research within medicine and interdisciplinary projects, reinforcing the need for open communication and collaboration among researchers.
Challenges of Data Sharing
Data sharing offers several opportunities, but it also presents notable challenges. These challenges must be addressed to promote effective data sharing practices in research. Among them, technical barriers and cultural resistance are critical issues that can hinder progress.
Technical Barriers to Access
Technical barriers to data sharing can manifest in various forms and can significantly impede collaboration. Firstly, inadequate infrastructure is a common issue. Not all institutions have the same level of technological resources and capabilities. Older systems might limit data format compatibility or slow down the sharing process. These limitations can frustrate researchers who need timely access to data.
Another aspect involves cybersecurity concerns. Sensitive data can be exposed during sharing, leading to potential breaches. Institutions often hesitate to share data openly due to fears about misuse or lapses in data protection. Strong security protocols are essential. Researchers and institutions must prioritize creating secure channels for data exchange, which can sometimes require additional investments.
Additionally, interoperability issues arise when different platforms and systems are employed. Diverse data formats and standards can complicate the merging of datasets. This situation often demands additional work, such as converting data or integrating multiple systems, which can be time-consuming and resource-intensive.
In summary, technical barriers necessitate careful consideration of infrastructure, security measures, and compatibility among systems. Addressing these issues effectively is vital for a smoother data-sharing experience that fosters collaboration.
Cultural Resistance within Disciplines
Cultural resistance can be a formidable barrier to data sharing. Different academic disciplines often have distinct norms and values regarding data usage and sharing. For instance, certain fields may prioritize proprietary approaches, leading to reluctance in sharing data. Researchers may view their findings as personal intellectual property, hesitant to make them available for public use.
Moreover, the lack of incentives to share data can contribute to this cultural resistance. Often, researchers are evaluated based on their individual output, resulting in a mindset that discourages collaboration. Without clear rewards or recognition for sharing data, many researchers may not prioritize it, limiting the potential for interdisciplinary collaboration.
Another issue lies in the social capital involved. Researchers might be concerned that sharing data could weaken their own position within their community. There's often a fear that open access might lead to misuse or misinterpretation of their work. The climate of mistrust can deter even the most well-intentioned individuals from data sharing, creating silos that restrict research advancement.
Addressing cultural resistance requires strategic initiatives. Institutions could develop robust policies that promote the value of data sharing while also providing incentives. Training and awareness programs can help change perceptions about the importance of sharing. Building a culture that prioritizes collaboration over competition is essential for overcoming these barriers.
"Overcoming cultural resistance requires strategic initiatives that promote the value of data sharing."
Future Directions in Data Sharing
The evolution of data sharing practices in research is pivotal to advancing scientific inquiry and collaboration. As we move forward, it is imperative to explore how emerging trends and new technologies can reshape the landscape of data accessibility and utilization. A clear understanding of future directions in data sharing holds significant benefits not only for researchers but also for institutions and society at large.
Emerging Trends in Data Sharing
Recent years have seen substantial shifts in how researchers approach data sharing. The integration of cloud computing and data management platforms has made remote access not just feasible but essential.
Some key trends to consider include:
- Increased Use of Open Data Platforms: Open data initiatives are gaining popularity as they allow researchers to share findings widely, encouraging collaboration and scrutiny. Platforms like the Harvard Dataverse and Zenodo are becoming more prominent.
- Advancements in Data Interoperability: As more researchers share their data, the need for standardized formats becomes critical. Technologies that promote interoperability facilitate better data sharing across disciplines.
- Role of Artificial Intelligence: AI is significantly changing the data analysis landscape. Machine learning algorithms can analyze shared datasets, providing new insights and making data-driven predictions.
- Enhanced Data Security Measures: As data sharing increases, so do concerns about privacy and security. Emerging technologies offer solutions to ensure that shared data remains safe, especially regarding sensitive information.
These trends signal a shift toward more dynamic, collaborative research environments where data is shared openly and responsibly.
Potential Impact on Scientific Research
The implications of these future directions in data sharing on scientific research are profound. When data is accessible, it can lead to more robust findings, the replication of results, and the faster discovery of innovative solutions to pressing problems. Potential impacts include:
- Accelerated Discoveries: Access to large datasets enables researchers to conduct analyses that were previously unfeasible. This can lead to breakthroughs in fields such as medicine, climate science, and social studies.
- Greater Collaboration: Researchers are likely to collaborate across boundaries, fostering interdisciplinary work. This leads to a more comprehensive understanding of complex issues that cannot be solved in isolation.
- Enhanced Public Trust: Transparent data sharing practices can build public trust in scientific findings. Open access to data allows for independent verification of results, bolstering credibility in the research community.
"Data sharing enhances innovation and collaboration, paving the way for groundbreaking research outcomes."
As we navigate these changes, it will be crucial for researchers, educators, and institutions to embrace these trends and adapt to the evolving data-sharing landscape. This proactive approach will help maximize the potential of shared data in scientific research.
Epilogue
Summary of Key Points
Throughout this article, we have examined several pivotal aspects of data sharing:
- Importance of Collaboration: Data sharing fosters collaboration, which leads to more robust results across disciplines.
- Ethical Considerations: Ethical practices surrounding data sharing, including informed consent and data privacy, are paramount in maintaining public trust.
- Technology's Role: Advances in technology, such as cloud platforms and blockchain, facilitate seamless data sharing while ensuring security and reliability.
- Policy Frameworks: Institutional guidelines and funding agency requirements play a crucial role in shaping data-sharing practices.
- Future Trends: Emerging trends indicate an increasing acceptance and integration of shared data in research, resulting in enhanced scientific discovery.
In synthesizing these elements, we can see that the collective efforts of the scientific community, along with well-defined policies and technological support, can lead to significant advancements in research outcomes.
Call to Action for Researchers and Institutions
As we look to the future, it is essential for researchers and institutions to commit actively to data sharing principles. Here are some actionable steps:
- Implement Data Management Plans: Develop comprehensive data management plans that outline how data will be shared and stored, ensuring compliance with ethical standards.
- Promote Open Data Initiatives: Encourage participation in open data initiatives within their disciplines, promoting transparency and collaboration.
- Foster a Culture of Sharing: Cultivate an organizational culture that values data sharing as a norm rather than an exception.
- Engage with Policy Makers: Work closely with policymakers to shape guidelines that promote effective data sharing while addressing ethical concerns.
- Invest in Training: Provide training for researchers on best practices for data sharing and management, thereby building capacity within the research community.
By actively engaging in these practices, researchers and institutions can leverage the power of shared data to advance scientific research significantly. This concerted effort will not only enhance the credibility of research but also contribute to the broader goal of improving collective knowledge in various fields.