Transformative Impact of AI in Healthcare Research
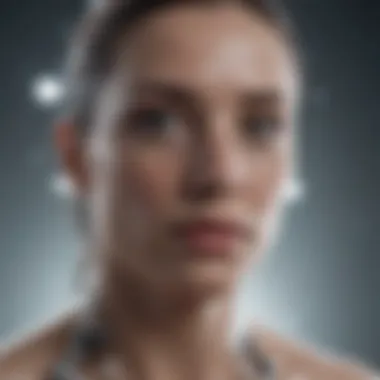
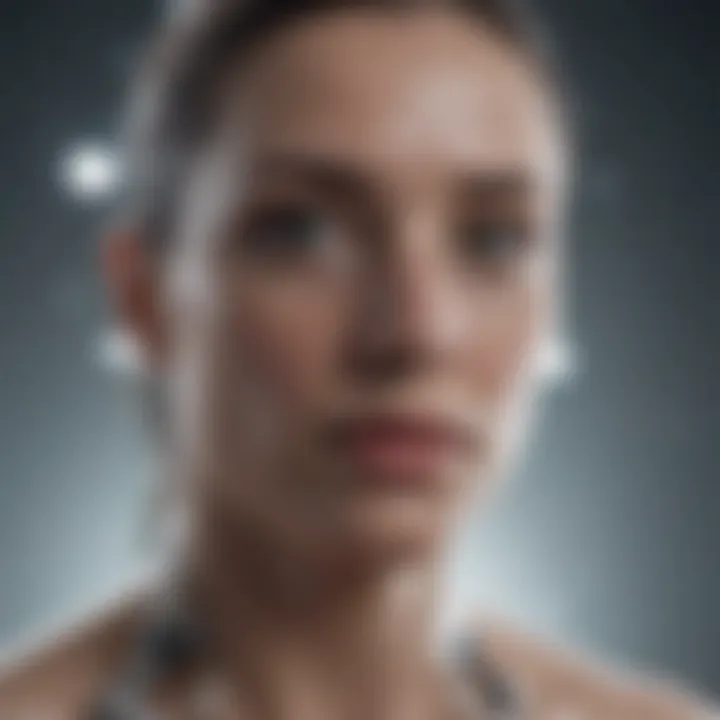
Intro
Artificial intelligence (AI) has become a cornerstone of innovation in various sectors, but its potential in healthcare research is particularly significant. The integration of AI technologies is accelerating the evolution of methodologies used in diagnostics, treatment predictions, and patient management. This article addresses how AI reshapes these facets, emphasizing benefits and challenges inherent in its application.
Despite its promising advantages, AI also raises ethical concerns and issues regarding data privacy. These complexities call for a nuanced understanding among students, researchers, educators, and professionals, who strive to harness AI's power while navigating these challenges.
Methodology
Overview of Research Methods Used
To uncover the role of AI in healthcare research, a diverse array of research methods have been employed. Quantitative studies have demonstrated statistical significance and predictive accuracy of AI algorithms in clinical settings. Meanwhile, qualitative analyses complement these findings by exploring the subjective experiences of healthcare practitioners who use these tools. Systematic reviews allow for comprehensive evaluations of existing literature, helping to synthesize varied perspectives and results.
Data Collection Techniques
Data collection in this realm involves several techniques, integrating both traditional and advanced methods. Some common approaches include:
- Clinical Trials: These trials often incorporate AI algorithms to monitor patient outcomes and treatment efficacy in real time.
- Surveys and Interviews: Engaging with healthcare professionals helps to gather insights on their perceptions and experiences with AI implementations.
- Big Data Analytics: Leveraging vast amounts of patient data allows for deeper insights into treatment outcomes and predictive modeling.
Data privacy is paramount; therefore, adherence to regulations such as HIPAA is crucial when handling sensitive patient information.
"AI can drastically improve decision-making in healthcare, but data privacy must not be compromised in the process."
Future Directions
Upcoming Trends in Research
As AI technology continues to develop, several intriguing trends are emerging in healthcare research. Machine learning and natural language processing are increasingly being used to analyze patient charts and unstructured data. Furthermore, there is a growing trend toward personalized medicine, where AI algorithms enhance treatment strategies tailored to individual patients' profiles.
Areas Requiring Further Investigation
Despite the advancements, numerous areas remain underexplored. Future research must address:
- The implications of AI in understanding complex diseases such as cancer.
- The ethical dimensions concerning algorithmic bias and accountability.
- Strategies for improving integration of AI tools in clinical workflows.
In summary, while AI offers substantial potential in healthcare research, understanding its limitations and challenges is vital for effective integration and utilization.
Prelude to Artificial Intelligence in Healthcare
Artificial Intelligence (AI) is a critical topic within the realm of healthcare research. It serves as a transformative force that is reshaping various aspects of medical practice and research methodologies. The integration of AI offers significant benefits that can enhance healthcare delivery, improve patient outcomes, and streamline research processes. Notably, AI can analyze vast amounts of data far beyond human capabilities, which allows for deeper insights and quicker decision-making.
AI technologies can assist in mitigating challenges faced in healthcare, such as resource limitations and increasing patient demands. The importance of AI lies not only in its applicability but also in its potential to revolutionize healthcare practices that have remained unchanged for decades. This section aims to delineate the foundational concepts of AI specifically in the context of healthcare.
Definition of Artificial Intelligence
Artificial Intelligence refers to the simulation of human intelligence in machines designed to think and act like humans. It encompasses a variety of techniques and technologies that enable computers to perform tasks that, when executed by humans, typically require cognitive functions such as learning, reasoning, and problem-solving. AI can be categorized into various types:
- Narrow AI: This type refers to AI systems that are designed for specific tasks, such as diagnosing diseases or managing patient schedules.
- General AI: Although this is more theoretical, General AI would possess the ability to perform any intellectual task that a human being can do.
In healthcare, AI employs machine learning algorithms, natural language processing, and other technologies to analyze complex data sets, leading to better patient diagnoses and treatment options.
Evolution of AI in Healthcare
The evolution of Artificial Intelligence in healthcare can be traced back several decades. It began with basic systems that could assist in administrative tasks, gradually progressing to more sophisticated applications in diagnostics and treatment recommendations.
In the early stages, AI was primarily limited to expert systems, which provided guided decisions through rule-based algorithms. However, with advances in computational power and data availability, machine learning, a subset of AI, has gained prominence.
Recent developments like deep learning and neural networks have propelled AI to several new heights. Today, AI is becoming integral to various areas:
- Medical Imaging: Algorithms are trained to detect abnormalities in imaging data with a precision that sometimes surpasses that of human experts.
- Predictive Analytics: AI can forecast patient outcomes by analyzing trends in historical data, which aids in timely interventions.
- Robotics: Robotics, powered by AI, is now increasingly utilized in surgeries and rehabilitation, improving precision and reducing recovery times.
This ongoing evolution underscores the need for continued research and investment into AI technologies, highlighting their critical role in enhancing healthcare research.
"The potential of AI in healthcare represents both a significant opportunity and a challenge for researchers and practitioners alike."
Through understanding the definition and evolution of AI, one can appreciate how it serves as a keystone in enhancing healthcare research and improving patient care.
AI Technologies in Healthcare
AI technologies are reshaping the landscape of healthcare by introducing novel tools and methodologies that enhance medical research and patient care. These technologies are pivotal in driving innovations that can lead to more accurate diagnostics, better treatment protocols, and improved patient outcomes. In this section, we will examine three major AI technologies: Machine Learning, Natural Language Processing, and Computer Vision. Each of these technologies plays a critical role in advancing healthcare research and practice.
Machine Learning
Machine Learning (ML) is a subset of artificial intelligence that allows systems to learn from data and improve their performance over time without explicit programming. In healthcare, ML algorithms are utilized for various purposes, including predictive modeling, risk assessment, and image analysis.
One crucial application is in clinical diagnostics. Machine learning models can analyze vast amounts of patient data to identify patterns that may indicate specific diseases or conditions earlier than traditional methods. For instance, algorithms can detect anomalies in medical imaging that radiologists might overlook, leading to timely interventions.
- Benefits of Machine Learning:
- Improved diagnostic accuracy
- Reduced time in data analysis
- Enhanced ability to spot trends in large datasets
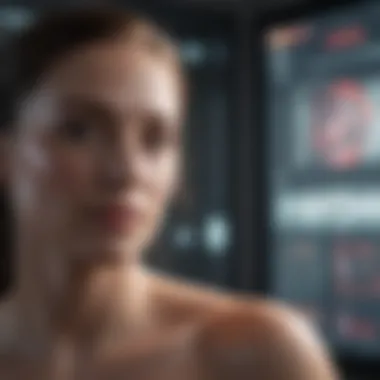
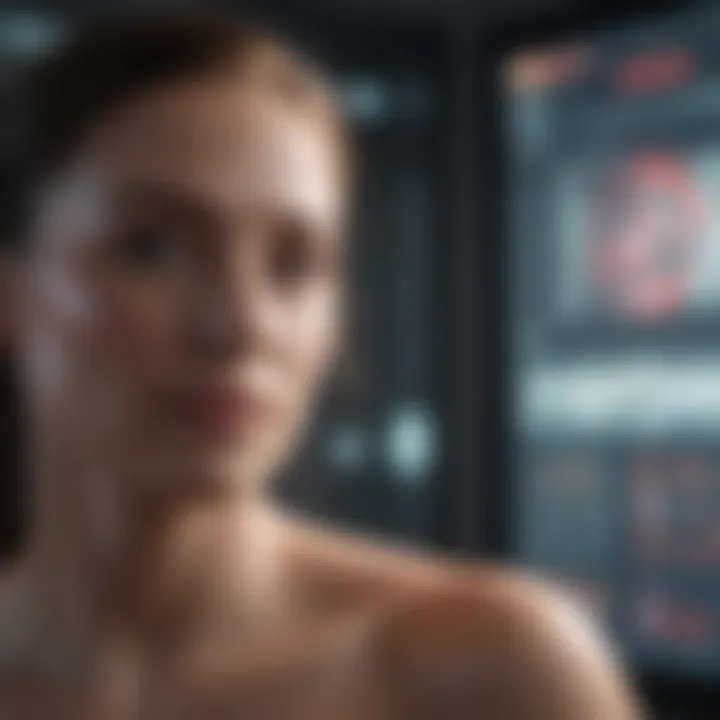
However, the adoption of machine learning also raises concerns. The necessity for high-quality data and the risk of algorithmic bias can impact the validity of outcomes. As machine learning becomes more integrated into healthcare, it's critical to address these issues to ensure equitable and effective patient care.
Natural Language Processing
Natural Language Processing (NLP) focuses on the interaction between computers and human language. In the context of healthcare research, NLP enables the extraction of valuable insights from unstructured data, such as clinical notes, research articles, and patient feedback.
- Applications of Natural Language Processing:
- Automated analysis of patient records
- Development of chatbots for patient interaction
- Extraction of information from scientific literature to assist in research
By utilizing NLP, researchers can gain a more comprehensive understanding of health trends, patient sentiments, and treatment effectiveness. This technology enhances the capacity for evidence-based medicine by summarizing vast quantities of literature rapidly. Despite its potential, NLP still faces challenges related to language nuances and contextual understanding, necessitating continuous advancements in its algorithms.
Computer Vision
Computer Vision is another pivotal AI technology employed in healthcare, particularly in the analysis of medical images. It involves the automatic extraction, analysis, and understanding of information from images. This technology is transforming fields such as radiology and pathology.
Here are some key uses of computer vision in healthcare:
- Medical Imaging: Algorithms can process X-rays, MRIs, and CT scans to detect abnormalities or diseases.
- Surgical Assistance: Real-time image analysis can assist surgeons by providing enhanced views during procedures.
- Monitoring Patient Conditions: Wearable devices with computer vision capabilities can track patient movements and monitor recovery progress.
The potential of computer vision lies in its ability to reduce human error and enhance precision in diagnosis and treatment. Nevertheless, similar to other AI technologies, ensuring data quality and addressing privacy concerns will be crucial as this technology evolves.
"AI technologies in healthcare have the potential to revolutionize patient care, but careful implementation is essential to avoid pitfalls."
Applications of AI in Healthcare Research
Artificial Intelligence has significantly changed how research is conducted within healthcare. The applications of AI systems in this field are broad and immensely impactful. These advancements not only enhance the quality of healthcare services but also streamline research processes, making them more efficient. The importance of this section lies in illustrating how AI transforms specific areas such as clinical diagnosis, predictive analytics, drug development, and personalized medicine. Understanding these applications is vital for students, researchers, educators, and professionals who aim to remain informed about the cutting-edge integration of technology in healthcare.
Clinical Diagnosis
AI plays a critical role in clinical diagnosis by providing tools that assist healthcare professionals in identifying medical conditions more accurately and promptly. Algorithms processing vast amounts of data can detect patterns that may be invisible to human eyes.
For instance, AI models analyzing medical imaging data can distinguish between healthy and diseased tissues. Technologies like deep learning have shown promising results in diagnosing conditions such as breast cancer and diabetic retinopathy. These advancements lead to improved patient outcomes due to early detection and treatment.
Moreover, AI enhances diagnostic precision, minimizing the risk of false negatives or positives, which can have serious consequences. By integrating AI into clinical practices, healthcare providers can optimize their diagnostic protocols, thus offering a more reliable service.
Predictive Analytics for Patient Outcomes
Predictive analytics, powered by AI, serves as a powerful instrument in anticipating patient outcomes. By analyzing historical health data, patient demographics, and clinical variables, AI systems generate models that can predict the likelihood of specific health events.
This capability is crucial for proactive patient management. For example, healthcare professionals can use AI to assess the risk of readmission for patients with chronic diseases. Understanding these risks helps tailor individualized treatment plans and interventions, ultimately reducing hospital readmissions and associated costs.
Furthermore, predictive analytics permits continuous monitoring of patient data, ensuring that necessary adjustments to treatment or care can be made in real-time.
Drug Discovery and Development
The drug discovery process is traditionally time-consuming and costly, often taking years before a new medication reaches the market. AI has emerged as a disruptive force throughout this process. By using predictive modeling, AI can accelerate the identification of potential drug candidates, significantly shortening the timeline from concept to clinical trial.
AI algorithms analyze chemical compounds and their interactions with biological targets. For instance, companies like Atomwise employ AI to simulate the interaction between drugs and biological systems, enabling them to prioritize candidates with the highest potential for success. This innovation reduces not only the costs but also the risks involved in drug development.
Ultimately, AI not only streamlines processes but also enhances the overall quality and efficacy of newly developed medications, ensuring they meet the patients' needs.
Personalized Medicine
Personalized medicine utilizes AI to tailor treatment based on individual patient characteristics. By processing extensive genetic data, AI can help identify which treatments are most effective for specific genetic profiles. This shift towards targeted therapies aligns with the goal of maximizing therapeutic efficacy while minimizing adverse effects.
With personal health data fed into sophisticated algorithms, healthcare providers can design treatment plans that are customized to each patient's unique needs, optimizing the entire care experience. For example, in oncology, AI tools can evaluate genetic mutations in tumor samples, guiding oncologists to choose therapies that are more likely to lead to successful outcomes.
By focusing on personalization, healthcare systems can deliver more effective treatments, improving patient satisfaction and overall health outcomes.
The integration of AI technologies into healthcare research is not just a trend but a paradigm shift that promises to redefine how healthcare is delivered, making it more precise and patient-centered.
Benefits of Integrating AI in Healthcare Research
Artificial Intelligence (AI) technology is increasingly recognized as a transformative force in healthcare research. Its integration brings various benefits that enhance not just accuracy but also efficiency in healthcare outcomes. The relevance of these benefits in this article is critical because they showcase how AI can fundamentally change research dynamics, leading to better patient care and optimized processes. As healthcare becomes increasingly data-driven, the role of AI becomes even more pivotal, forming a bridge between vast amounts of information and actionable insights.
Enhanced Diagnostic Accuracy
One of the key advantages of AI in healthcare research is the enhancement of diagnostic accuracy. Machine learning algorithms are capable of analyzing large datasets with incredible precision. This capability allows for improved prediction and identification of diseases, often at earlier stages than human practitioners could achieve. For instance, AI systems have shown remarkable proficiency in analyzing medical imaging, such as MRIs and CT scans. By utilizing deep learning techniques, these systems can detect anomalies that might be missed by human eyes.
The importance of accuracy cannot be overstated. Misdiagnosis or delayed diagnosis can have profound impacts on treatment outcomes, patient satisfaction, and overall healthcare costs. AI's ability to provide reliable diagnostic assistance can result in fewer errors, leading to better patient outcomes and optimized resource allocation. Moreover, as AI systems learn from new data, their diagnostic capabilities continue to improve, indicating an ongoing evolution in effectiveness.
Increased Efficiency in Research Processes
AI also contributes significantly to increased efficiency in healthcare research processes. Traditional research methods often involve time-consuming manual data collection, analysis, and interpretation. AI automates many of these tasks, helping researchers to focus their efforts on interpreting results rather than gathering data. For example, natural language processing can sift through massive volumes of scientific literature, pulling relevant insights quickly. This efficiency accelerates the research timeline, from hypothesis formulation to publication.
The ability to process vast amounts of data rapidly leads to quicker decision-making.
- Faster data analysis: Algorithms can analyze patient data and outcomes faster than traditional methods.
- Resource optimization: Reduced administrative workload allows healthcare professionals to redirect their focus toward patient care.
- Collaborative potential: Enhanced efficiency promotes collaborative studies, as findings can be readily shared and analyzed by other researchers.
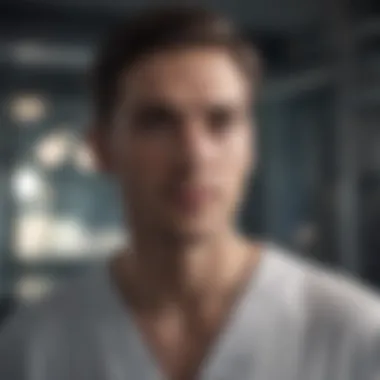
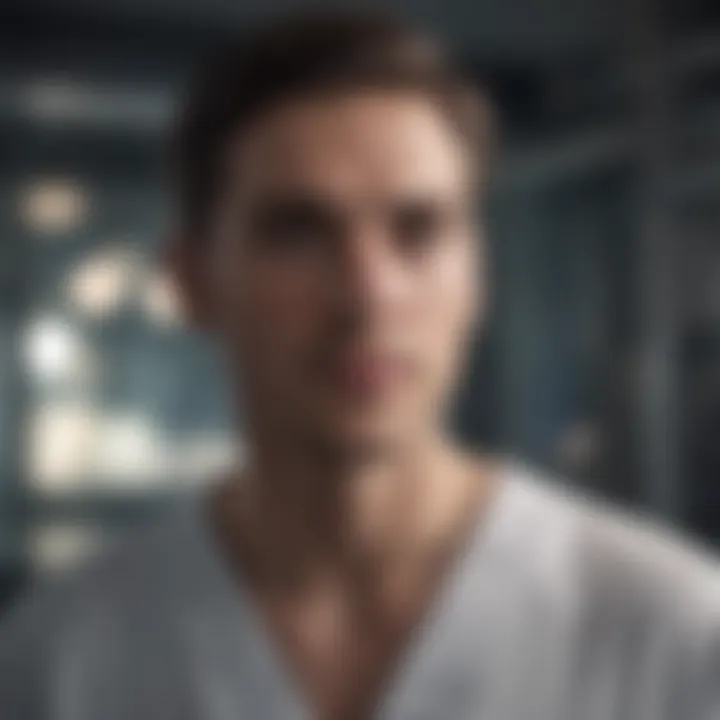
Additionally, AI systems can facilitate real-time monitoring of ongoing studies, allowing researchers to adjust variables based on immediate feedback. This adaptability is crucial for maintaining the integrity of research while ensuring that interventions remain relevant and effective.
In summary, integrating AI into healthcare research yields invaluable benefits such as enhanced diagnostic accuracy and increased efficiency in research processes. These elements are essential not only for improving patient care but also for driving innovation within healthcare research.
Challenges in Implementing AI in Healthcare Research
The integration of artificial intelligence into healthcare research is a meaningful development aimed at enhancing patient outcomes and improving clinical efficiencies. However, alongside the potential benefits lies a spectrum of challenges that can impede progress. Focusing on challenges in implementing AI in healthcare research is crucial for understanding the barriers as well as the strategies needed to effectively leverage these technologies. Key considerations include data privacy concerns, ethical implications, and the technical complexities involved in integrating AI systems into existing healthcare infrastructures.
Data Privacy Concerns
In healthcare, patient data is highly sensitive. The deployment of AI technologies often requires access to large datasets containing personal health information. This raises significant data privacy concerns. Ensuring that AI systems comply with privacy regulations, such as the Health Insurance Portability and Accountability Act (HIPAA), is vital. Organizations must navigate complex legal frameworks governing data collection and use.
Moreover, the risk of data breaches or misuse of information makes stakeholders uneasy. Implementing robust data protection mechanisms is essential to alleviate these fears. Encryption techniques and secure data storage methods should be standard practices. Moreover, implementing strict access controls will help safeguard against unauthorized data access.
Ethical Considerations
The ethical landscape surrounding AI in healthcare is intricate and warrants serious examination. One primary concern involves algorithmic bias. AI systems can inadvertently perpetuate existing biases if they are trained on skewed datasets, leading to unequal treatment outcomes across different patient groups. This problem underscores the importance of employing diverse data sources to ensure that AI algorithms are fair and equitable.
Furthermore, the transparent use of AI in medical decision-making raises important questions about accountability. Clinicians may hesitate to rely on AI-generated insights if they do not fully understand how those insights were derived. Establishing clear guidelines on the interpretability of AI tools is necessary to foster trust among healthcare professionals.
"A thoughtful approach to both privacy and ethics must be a foundational principle in developing AI technologies for healthcare."
Additionally, the issue of informed consent remains a challenge. Patients should be aware of how their data is being utilized and the implications of AI-driven decisions in their care. Balancing the innovative potential of AI with ethical responsibility is imperative in fostering public confidence in these technologies.
Overall, addressing these challenges requires a multifaceted approach that includes ongoing dialogue among stakeholders, effective policy-making, and continuous monitoring of AI systems in practice. By prioritizing data privacy and ethical considerations, the healthcare sector can better navigate the complexities of integrating AI into research.
Current Trends in AI Healthcare Research
The advancement of artificial intelligence is rapidly reshaping the landscape of healthcare research. There are several key trends currently evident that warrant attention. Understanding these trends is crucial because they not only reflect the evolving capabilities of AI but also highlight significant implications for patient care and medical practices. With the integration of AI in healthcare, organizations aim for improved health outcomes, patient engagement, and increased efficiency. This section explores two major trends in AI healthcare research: AI-driven health monitoring and telemedicine enhancements.
AI-Driven Health Monitoring
AI-driven health monitoring represents a revolutionary shift in how patient health can be tracked continuously. By employing machine learning algorithms and real-time data analytics, clinicians can observe patients' vital signs, lifestyle habits, and even behavioral patterns closely. This constant monitoring enables healthcare providers to identify anomalies and intervene earlier than traditional methods would allow.
- Benefits: The immediate health benefits from AI-driven monitoring include faster detection of issues that may escalate into serious health crises. For example, wearable technology can alert both patients and doctors to irregular heartbeats or other concerning metrics.
- Data Collection: The health data collected through these systems tends to be vast and varied. From heart rates to sleep patterns, the depth of information can lead to more personalized treatment strategies tailored to individual needs.
- Challenges: Data privacy continues to be a concern. Protecting sensitive patient information while ensuring the efficacy of these monitoring systems is a challenge that must be addressed.
"AI-driven health monitoring empowers clinicians to make informed decisions, leading to improved patient care."
Telemedicine Enhancements
Telemedicine has transformed from a supplemental service into a central component of healthcare delivery, particularly highlighted by the global pandemic. AI plays a pivotal role in enhancing telemedicine by providing tools that improve patient access and the quality of care.
- Virtual Consultations: One prominent feature of telemedicine is video consultations. AI technologies streamline this process, from scheduling appointments to providing virtual assistants that triage patient inquiries before they meet with healthcare professionals.
- Diagnostic Support: Advanced AI systems can assist in diagnosing conditions during telehealth visits. For instance, natural language processing can analyze patient symptoms described in conversations, providing healthcare providers with additional context to aid their clinical judgment.
- Broader Reach: By utilizing AI, telemedicine can extend its reach particularly in remote areas, where access to specialists is often limited. This fosters a more equitable healthcare landscape.
In summary, the current trends in AI healthcare research spotlight significant advancements that not only improve operational efficiency but also aim to enhance patient experiences and outcomes. As these technologies continue to evolve, the healthcare system may witness more profound transformations.
Future Directions of AI in Healthcare Research
The future of artificial intelligence in healthcare research is a topic of great significance. It points towards enhanced methodologies, improved patient outcomes, and a more efficient healthcare system overall. As technology continues to advance, AI won't just be integrated; it will become a cornerstone of medical research and practice. Understanding future directions can help stakeholders navigate the complexities of AI implementation along with maximizing its potential benefits.
AI for Public Health Management
AI tools have the power to transform public health management. Through data analysis and predictive modeling, AI can assist in tracking disease outbreaks and optimizing resource allocation. For example, algorithms can process vast amounts of data to identify patterns and predict future public health trends. This capability is vital in developing timely interventions, particularly in response to pandemics.
AI applications, such as machine learning models, can analyze social media trends and other real-time data sources to predict the spread of diseases. This proactive approach can lead to swift action plans, improving preparedness and responsiveness.
Moreover, AI can optimize vaccination strategies. By assessing factors like demographics, previous health data, and geographical information, AI can identify priority populations for vaccination campaigns. Effective public health is reliant on strategic planning, and AI proves advantageous in creating data-driven approaches.
Collaborative Research Initiatives
Another promising direction for AI in healthcare research is collaborative initiatives. Partnering across institutions, disciplines, and even borders can yield significant advantages. These collaborative efforts can produce comprehensive datasets, facilitating robust research that individual organizations might not be able to achieve alone.
Such partnerships could involve academia, healthcare providers, and technology firms. For instance, universities can work with AI companies to explore novel algorithms that improve diagnostic tools. Hospitals might collaborate with researchers to test AI models in real-world settings, ensuring that technological advances actually improve patient care.
"Collaboration is essential for leveraging AI's full potential in healthcare."
Additionally, funding agencies and governmental organizations can play an instrumental role by supporting transdisciplinary research initiatives. They can offer grants to research teams that aim to develop AI solutions addressing complex healthcare challenges. By pooling resources and expert knowledge, stakeholders can undertake more extensive studies, potentially leading to breakthroughs in treatment and patient care.
In summary, the future of AI in healthcare research encompasses significant advancements in public health management through data-driven decision making and the potential of collaborative initiatives to elevate research to new heights. These evolving trends showcase a shift in focus towards leveraging technology for maximum efficiency and efficacy in healthcare.
Real-World Case Studies
Real-world case studies in healthcare research provide practical examples of how artificial intelligence enhances medical practices and outcomes. These studies offer valuable insights into the application, effectiveness, and scalability of AI technologies. They serve as a bridge between theoretical frameworks and clinical practice, demonstrating AI's potential in improving patient care, diagnostic accuracy, and treatment efficiency.
Through various case studies, it becomes evident how AI can lead to tangible benefits, such as more accurate diagnoses, personalized treatment plans, and better management of healthcare resources. These examples not only highlight successful implementations but also showcase the challenges encountered and how they were addressed. The lessons learned can inform future initiatives and guide researchers and practitioners in AI integration within healthcare.
AI in Radiology
Radiology is one area where AI has made significant strides. Recent advancements in deep learning algorithms allow for improved image analysis, resulting in faster and more accurate interpretations of medical scans. For example, algorithms developed by Google Health and Stanford University have demonstrated high levels of accuracy in diagnosing conditions like pneumonia from chest X-rays.
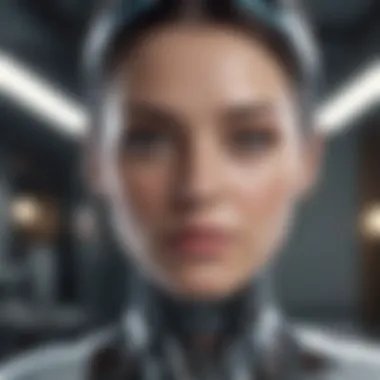
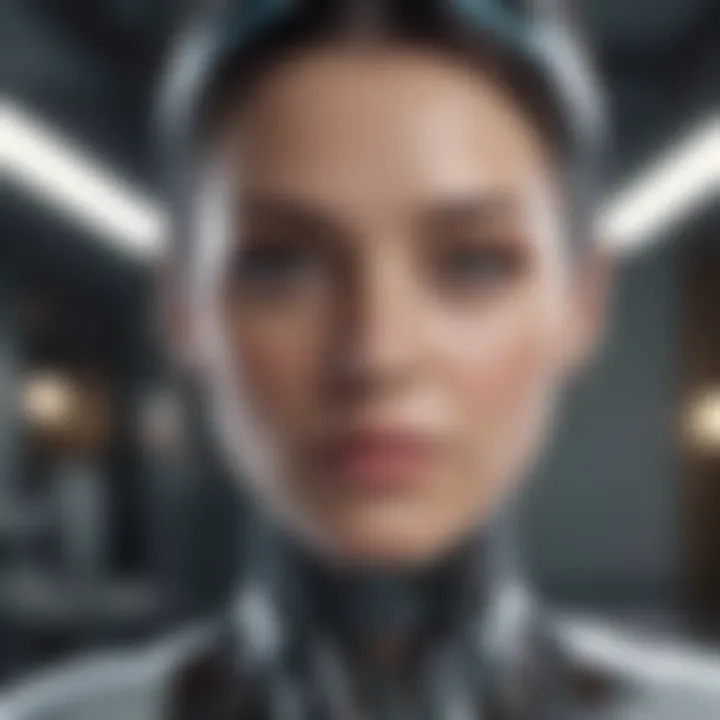
These AI systems can analyze thousands of images quickly, thus supporting radiologists in their decision-making processes. This is crucial in busy hospital settings where time is of the essence. The integration of AI can reduce the workload on radiologists, allowing them to focus on complex cases that require human judgment.
Besides efficiency, AI in radiology also enhances diagnostic capabilities, particularly in detecting subtle anomalies that might be overlooked by the human eye. The potential to lower false positive rates through AI adds another layer of trust in diagnostic processes, ultimately benefiting patient outcomes. However, continuous evaluation and validation are essential to ensure these AI systems maintain high standards of reliability in clinical settings.
AI Applications in Oncology
Oncology is another domain where AI has shown promise. The field of cancer research and treatment involves analyzing vast amounts of data, including imaging, genomic information, and patient demographics. AI systems can process and analyze this data rapidly, identifying patterns that may not be apparent to clinicians.
For instance, IBM Watson for Oncology has used AI algorithms to analyze patient records and provide treatment recommendations based on similarity to historical cases. This approach enables oncologists to tailor therapies specifically to individual patient profiles, improving the likelihood of positive outcomes.
Additionally, AI can enhance drug discovery by sifting through large datasets to identify potential drug candidates more efficiently, thereby reducing the time and cost associated with bringing new cancer therapies to market.
"AI applications in oncology are transforming the way we approach cancer treatment, setting the stage for more precise, personalized care."
Challenges do exist, such as the need for rigorous testing to ensure safety and efficacy, as well as ethical considerations concerning patient data use. Despite these hurdles, real-world case studies in oncology highlight the vast potential of AI to reshape cancer care, making it a critical area of focus for future research and investment.
Funding and Investment in AI Health Research
Understanding the funding and investment landscape in AI health research is essential for realizing the full potential of artificial intelligence in this field. The success of AI technologies in healthcare largely depends on the financial resources available for research and development. Investment in this area can enhance innovation, driving forward breakthroughs that can lead to improved patient care and better health outcomes. The significance of funding can be seen in various aspects such as the development of new health technologies and the ability to scale existing solutions.
Funding allows researchers to conduct extensive studies and clinical trials, gathering the necessary data to validate AI applications. This data is crucial for creating AI algorithms that are accurate and reliable. Furthermore, adequate financial support can lead to the formation of public-private partnerships that can accelerate advancements in healthcare technologies.
Federal funding plays a crucial role in promoting research initiatives. Governments are increasingly recognizing the importance of investing in AI health research to tackle significant public health issues. These initiatives often focus on disease prevention, diagnostic research, and healthcare efficiency. By prioritizing AI research, governments can better prepare their healthcare systems for future challenges, including aging populations and the rising prevalence of chronic diseases.
- Research Grants: Governments often allocate substantial grants to research institutions and universities, enabling them to explore innovative AI applications.
- Public Health Programs: Various government programs specifically aim to leverage AI for improving population health, signifying a strategic commitment to research funding.
However, while government funding is essential, private sector investments also hold a significant contribution. Companies in the healthcare and technology sectors are increasingly investing their resources into advancing AI capabilities. This not only fosters competition, driving innovation but also aids in establishing a comprehensive ecosystem for health research.
Government Initiatives
Government initiatives play a pivotal role in the sustainability and growth of AI health research. One notable aspect includes the establishment of organizations that focus solely on the funding of AI-related projects in healthcare. The National Institutes of Health (NIH) and the Food and Drug Administration (FDA) in the United States are exemplary instances of entities that are continuously promoting AI research through funding.
Government-funded programs often target specific diseases or issues, motivating researchers to innovate solutions that are impactful.
- Strategic Partnerships: Collaborative efforts between healthcare organizations and governmental bodies can provide resources and support for research initiatives. Government funding often encourages universities and research institutions to partner with private firms, leading to a synergistic effect that amplifies research outcomes.
- Incentives for Research: Governments often introduce tax incentives or other financial benefits to encourage private companies to invest in AI health research. This collaborative approach has shown to yield promising results in various healthcare applications, including drug discovery and personalized medicine.
Private Sector Investments
Private sector investments have become increasingly important in the realm of AI health research. These investments are often led by technology firms, startups, and established pharmaceutical companies that recognize the transformative potential of AI.
Investments from private firms can drive the development of innovative solutions and technologies that directly address challenges faced in healthcare systems worldwide.
- Venture Capital: A considerable portion of funding for AI startups in the healthcare field comes from venture capital. This type of funding is critical for fostering innovation and allowing startups to experiment with new ideas.
- Company Research Initiatives: Large corporations like Google, IBM, and Siemens invest heavily in AI research. They employ teams of data scientists and researchers to develop AI solutions tailored for healthcare, significantly accelerating the pace of technology advancement.
"Investment and funding in AI health research are not just about money. They represent a commitment to better health and innovative solutions that can transform patient care."
This collaborative effort creates a more robust framework for tackling the complex challenges within the healthcare sector.
Regulatory Framework for AI in Healthcare
The regulatory framework guiding the use of artificial intelligence in healthcare is imperative for the safe and effective deployment of these technologies. As AI solutions are increasingly integrated into clinical settings, the need for clear regulations becomes crucial. The framework ensures that AI-driven tools adhere to safety standards and promote patient welfare, addressing potential risks associated with their use. This section delves into key guidelines and compliance measures that characterize the regulatory landscape for AI in healthcare.
Guidelines for AI Use in Clinical Settings
Guidelines serve as a compass for healthcare practitioners and organizations in implementing AI technologies. In clinical settings, effective guidelines encompass the following aspects:
- Transparency: AI algorithms must clearly disclose how they derive conclusions and suggestions. This enables healthcare professionals to trust the recommendations provided by AI systems.
- Patient Safety: AI applications should be tested rigorously to showcase their reliability in diagnosing and treating conditions. Safety protocols must be in place to manage any incidents arising from AI misjudgments.
- Data Integrity: The data used by AI systems must be from reputable sources, and robust mechanisms should exist to maintain data accuracy over time.
- Informed Consent: Patients need to be aware of how AI technologies will be used in their treatment. Providing proper education builds trust and guardianship of patient rights.
These guidelines aim to create an ecosystem where AI enhances clinical decision-making without compromising patient care and safety.
Compliance and Standards
Compliance with regulatory standards is fundamental to ensuring the responsible use of AI in the healthcare industry. Key compliance elements include:
- Adherence to Local Regulations: Healthcare organizations must be aware of and conform to rules set by local governing bodies. This ensures that AI tools meet the legal requirements for operation.
- Standardization of Procedures: Developing standardized protocols for evaluating AI systems helps mitigate risks associated with technology deployment. This framework aids healthcare practitioners in uniformly assessing AI capabilities.
- Regular Audits: Periodic audits of AI performance and compliance with the established guidelines are essential. This process can help identify areas for improvement and ensure ongoing alignment with accepted standards.
- Training and Education: It is critical for medical staff to receive training on AI applications and corresponding regulations. Knowledgeable staff can better utilize these tools while adhering to compliance standards.
End
The conclusion serves as a vital component of this article, encapsulating the essential insights into the role of artificial intelligence (AI) in healthcare research. It is essential to synthesize the critical findings discussed throughout the various sections, allowing the reader to grasp the overarching narratives that illustrate AI's transformative power within this domain.
Summarizing Key Findings
In reviewing the influence of AI technologies on healthcare research, several key points emerge:
- Enhanced Diagnostic Accuracy: AI systems, especially through machine learning and computer vision, have significantly improved the precision of clinical diagnoses. These systems analyze vast datasets, identifying patterns that may not be visible to human practitioners.
- Predictive Analytics: AI's ability to predict patient outcomes based on historical data has revolutionized patient management strategies. This capability enables healthcare professionals to intervene earlier and personalize treatment plans more effectively.
- Drug Discovery Efficiency: AI has accelerated the drug discovery process, minimizing time and resources traditionally needed for research and development. By predicting how different compounds might interact biologically, breakthroughs can occur more swiftly.
- Ethical and Privacy Considerations: With the advancement of AI, ethical challenges and data privacy issues have arisen. Balancing innovation with ethical standards is paramount to ensure patient trust and safety.
These findings illustrate the depth of AI's impact and underline the necessity for ongoing research and regulatory vigilance.
Call for Future Research
Future research must center on several areas to further integrate AI into healthcare effectively. Some potential avenues include:
- Longitudinal Studies: Conducting extensive studies over time can provide insights into AI technology's long-term effects on patient outcomes and healthcare processes.
- Interdisciplinary Collaboration: Encouraging collaborations between technologists and healthcare professionals will help develop innovative solutions tailored to clinical needs.
- Ethical Framework Development: As AI becomes more ubiquitous, establishing comprehensive ethical guidelines will be necessary to navigate the complex landscape of data usage and patient interaction.
- Regulatory Impact Assessment: Evaluating how regulations evolve in response to AI advancements is crucial. Research should focus on compliance measures that protect patients while fostering innovation.
In summary, concluding this article reinforces the need for a balanced approach to AI in healthcare research, where advancements are pursued in tandem with ethical considerations and regulatory frameworks.