In-Depth Models in Scientific Research Analysis
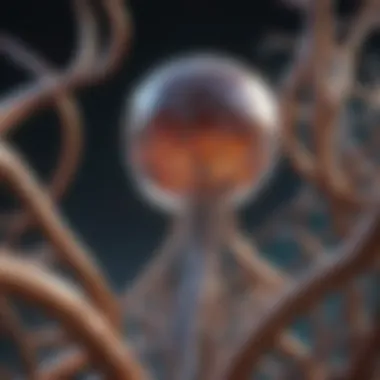
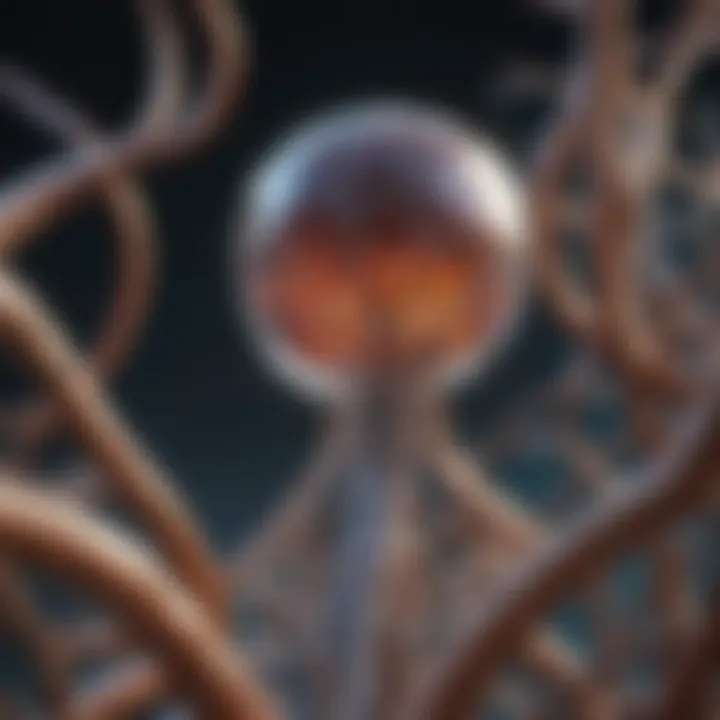
Intro
The importance of scientific models cannot be overstated. In various scientific disciplines, from biology to physics, models serve as fundamental tools. They allow researchers to simplify complex systems, anticipate outcomes, and draw conclusions based on empirical data. Understanding these models is essential for students, researchers, and professionals who aim to contribute meaningfully to their fields.
This article delves into major models across scientific domains. It highlights their roles, limitations, and impact on research advancements. By dissecting each model, the intricacies and nuances become clearer. The discussion will also explore interdisciplinary approaches that enhance collaboration, which is increasingly necessary as scientific questions grow in complexity.
By the end of this exploration, the audience will possess a comprehensive understanding of scientific models. This includes their applications and implications for future research. It will also illustrate how these models aid in interpreting complex phenomena, ultimately promoting advancements in diverse scientific fields.
Methodology
Understanding the methodologies behind scientific models is crucial for evaluating their effectiveness and applicability.
Overview of research methods used
The research methods employed in studying models vary. Common approaches include:
- Experimental research: Involves manipulation of variables to establish cause-effect relationships. This method is prevalent in disciplines like chemistry and biology.
- Computational modeling: Utilizes mathematics and algorithms to simulate scenarios. It's essential in fields such as physics and earth sciences. Researchers can analyze interactions within a system without physical experimentation.
- Theoretical analysis: Involves developing theoretical frameworks that predict behaviors. This method often complements empirical studies, especially in social sciences.
Data collection techniques
Data collection plays a pivotal role in the validity of scientific models. Some common techniques include:
- Surveys: Used to gather large amounts of data from participants, helping validate models in social sciences.
- Field studies: Researchers gather data from natural settings, providing context to theoretical models in ecology and geology.
- Laboratory experiments: Controlled environments ensure precision, crucial for chemical and biological modeling.
Future Directions
As scientific fields evolve, so do the models that support them. Future research will likely reveal new trends and areas that require further investigation.
Upcoming trends in research
- Interdisciplinary collaboration: There is a growing trend to integrate knowledge from different scientific areas. For example, combining biology and technology leads to advancements in bioinformatics.
- Increased use of AI and machine learning: These technologies help refine models by processing vast data sets, offering new perspectives and predictive power.
- Focus on sustainability: As global challenges arise, models that address ecological issues will gain prominence. This includes climate modeling and resource management frameworks.
Areas requiring further investigation
- Limitations of existing models: Many current models fall short in addressing real-world complexities. This presents an opportunity for researchers to refine or develop new models that better capture intricate interactions.
- Ethical implications of modeling: With advancements come ethical questions regarding data usage and model implications in policy decisions. Understanding these aspects is essential for responsible research.
In summary, the future of scientific modeling indicates a combination of technological innovation and interdisciplinary collaboration. It will likely reshape how research is conducted and influence the development of new models that address complex scientific challenges.
Foreword to Scientific Models
Scientific models are essential tools that facilitate our understanding of complex phenomena across various disciplines. In the realm of scientific research, models serve as simplified representations of reality, enabling researchers to hypothesize, test, and refine their ideas. Such models can be conceptual or mathematical, and their development is integral for advancing knowledge in fields such as biology, chemistry, physics, and earth sciences.
The importance of scientific models lies in their ability to distill intricate systems into more manageable forms. They help researchers to visualize processes, predict outcomes, and interpret data that might otherwise seem bewildering or inaccessible. Moreover, models support the iterative nature of scientific inquiry. As new data emerges or as technological advancements occur, models can be adjusted, expanded, or even entirely restructured. This adaptability underscores the dynamic relationship between models and the knowledge they represent.
Definition and Relevance
A scientific model can be defined as a theoretical construct that represents specific aspects of reality. It can vary from a simple diagram illustrating a concept to complex mathematical equations delineating interactions within a system. The relevance of these models is multifaceted. They not only assist in validating hypotheses but also provide frameworks within which scientists can collaborate and innovate.
Models also have practical applications. For instance, in biology, the cell theory model offers insight into the fundamental unit of life. In contrast, in climate science, models are crucial for predicting future climate patterns and informing policy decisions. Thus, understanding models is pivotal to grasping the broader scientific narrative and the implications of research findings.
Historical Context of Scientific Modeling
The historical context of scientific modeling is rich and revealing. From ancient civilizations to modern-day science, models have evolved alongside human understanding of the natural world. For instance, the early representations of the solar system—such as Ptolemy's geocentric model—were pivotal in furthering astronomical studies. These early models, despite their inaccuracies, provided a framework for systematically observing celestial bodies.
The transition to the heliocentric model proposed by Copernicus marked a significant shift in thinking and illustrated the role of models in challenging prevailing paradigms. Similarly, the development of atomic models by Dalton, Thomson, and Rutherford showcased how cumulative understanding leads to more refined representations of matter.
In contemporary research, models have become increasingly sophisticated, incorporating advanced computational techniques and interdisciplinary approaches. This historical journey not only highlights the evolution of scientific inquiry but also emphasizes the necessity of models in shaping our understanding of the universe.
"Models are not merely tools; they are lenses through which we examine the complexities of our world."
Understanding major models in scientific research is not only an academic exercise; it is a gateway to unlocking the intricacies of nature and advancing the frontiers of knowledge.
Major Models in Biology
Biology is a field rich with complexity and intricacies in which various models play a crucial role. The models established in biology help in understanding living systems, the interrelation of organisms, and the mechanism by which life itself functions. By providing structured frameworks, these models allow for predictions, hypothesis testing, and the resolution of biological questions.
The benefits of studying major models in biology encompass not only a deeper comprehension of life sciences but also advancements in areas such as medicine, ecology, and biotechnology. They serve as blueprints, guiding research direction and influencing experimental design. Nonetheless, researchers must consider the limitations of these models. Each model has its scope and can sometimes oversimplify complex processes, which necessitates a critical analysis.
In this section, we explore specific significant models within biology. We begin with the foundational Cell Theory, then examine evolutionary models that have shaped our understanding of species development, and finally, delve into genetic models that explain hereditary traits.
Cell Theory
Cell Theory is a fundamental concept in biology that posits all living organisms are composed of cells. This model established three primary tenets: 1) All living things are made up of cells, 2) The cell is the basic unit of life, and 3) All cells arise from pre-existing cells.
The importance of Cell Theory cannot be overstated. It provides a unifying framework that underpins all biological research, allowing scientists to approach the study of life from a cellular level. The formulation of this theory facilitated advances in fields such as microbiology, genetics, and histology.
Given its comprehensive nature, Cell Theory is essential in both educational and research contexts. This model encourages a focus on cellular processes, which are crucial for understanding health, disease, and development in living organisms.
Evolutionary Models
Evolutionary models offer insights into how species change over time through various mechanisms. These models help explain the diversity of life on Earth and are pivotal for understanding the principles of natural selection, adaptation, and speciation.
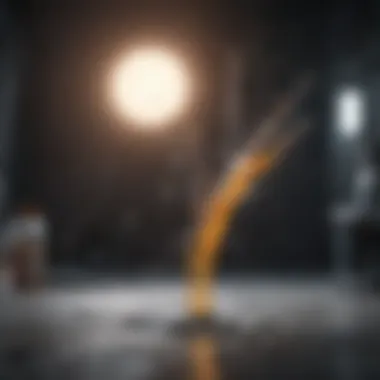
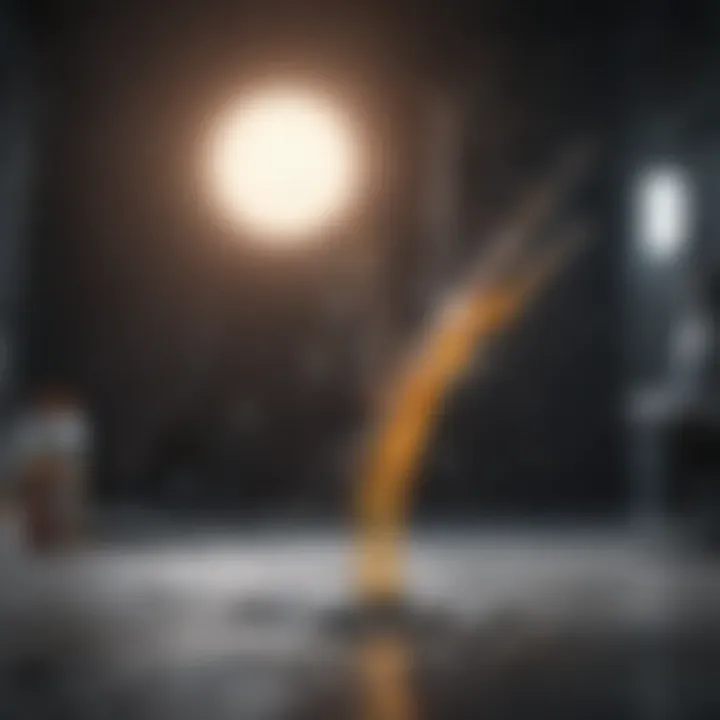
Darwinian Evolution
Darwinian Evolution, articulated by Charles Darwin, is centered around the concept of natural selection. This mechanism describes how traits that enhance survival and reproduction tend to persist in a population over generations.
A core characteristic of Darwinian Evolution is its emphasis on the environmental pressures that shape species. This model is popular due to its empirical support and relevance in various biological disciplines, from ecology to genetics. What sets Darwinian Evolution apart is its ability to predict patterns of species distribution and adaptation.
However, it is vital to acknowledge some disadvantages, such as the oversimplification of evolutionary processes. Critics argue that it might not capture the complete picture of how evolution operates at the molecular level.
Lamarckism
Lamarckism proposes that organisms can pass on traits acquired during their lifetime to their offspring. While largely discredited, this model provides an interesting perspective on the study of evolutionary biology.
A key characteristic of Lamarckism is its focus on the influence of the environment on trait development. It remains a notable choice for historical discussions within evolutionary biology. Its unique proposition that organisms could adapt within their lifetimes serves as a contrast to Darwin's model.
However, the model faces significant criticism for lacking empirical evidence and mechanisms. Its shortcomings lie in the inability to account for genetic inheritance as understood in modern biology.
Modern Synthesis
The Modern Synthesis integrates Darwinian evolution with Mendelian genetics, providing a comprehensive view of evolutionary processes. This model highlights the role of genetic variation and population genetics in evolution.
A defining aspect of the Modern Synthesis is its rigorous scientific grounding. This model synthesizes various biological concepts, making it a robust choice for understanding evolutionary biology today. Its strength lies in its ability to unify diverse disciplines and explain phenomena through a genetic lens.
Nevertheless, some argue that it may not sufficiently address elements like gene-culture coevolution or the influence of epigenetics, which could enhance our understanding of evolution.
Genetic Models
Genetic models are essential to understanding heredity and variations. They provide frameworks for examining how traits are passed down through generations and how genetic mutations can influence populations. These models help clarify the underlying mechanisms of disease, trait expression, and evolutionary changes.
The examination of genetic models enriches our grasp of both historical and contemporary biological issues. They pave the way for advancements in genetics, genomics, and biotechnologies, thereby significantly impacting medicine and agricultural sciences.
Overall, each model within biology serves as a building block for scientific inquiry. Through these models, we gain a clearer picture of life processes, paving the way for future discoveries.
Major Models in Chemistry
The study of chemistry is deeply intertwined with the models that help us understand the nature of matter. Major models in chemistry seek to explain various phenomena, ranging from atomic structure to chemical reactivity. These models serve as essential tools for chemists and play a significant role in both academic research and practical applications. Through these models, scientists can predict outcomes of chemical reactions and understand interactions at the molecular level. Their influence stretches across various fields, including materials science, pharmacology, and environmental science.
Atomic Models
Dalton's Model
Dalton's Model is one of the foundational concepts in chemistry, developed in the early 19th century. This model introduces the idea that each element is composed of tiny, indivisible particles called atoms. One key characteristic of Dalton's Model is its simplicity, which allows it to be easily understood and conveyed. It posits that atoms of the same element are identical in mass and properties, while those of different elements vary. This model laid the groundwork for modern atomic theory and is beneficial within this article because it provides a clear starting point for understanding more complex models that followed.
A unique feature of Dalton's Model is its focus on whole-number ratios in the formation of compounds. This characteristic highlights the concept of the conservation of mass, which continues to be a fundamental principle in chemistry. However, a disadvantage is that it does not account for isotopes, which contain atoms of the same element with different masses.
Thomson's Plum Pudding Model
The Plum Pudding Model, proposed by J.J. Thomson in 1904, suggests that atoms are a mix of positively charged 'pudding' with negatively charged electrons scattered throughout it, resembling a plum pudding. Its significance lies in introducing the concept of electrons as components of the atom. The model is particularly notable because it was one of the first to identify the electron, marking a pivotal moment in atomic theory.
One key characteristic of the Plum Pudding Model is that it portrays the atom as a uniform distribution of charge. This model is popular for illustrating how electrons are embedded in the atom, and it reflects the experimental evidence of the time. However, the model's failure to explain atomic stability and its inability to account for the nucleus revealed limitations.
Rutherford's Model
Ernest Rutherford's Model emerged from his gold foil experiment in 1909. It claimed that atoms consist of a small, dense nucleus surrounded by orbiting electrons. This new perspective was revolutionary, as it established the nuclear structure of the atom. A fundamental characteristic of Rutherford's Model is the dense nucleus, which contains protons and, later discovered, neutrons.
This detail makes Rutherford's Model a beneficial choice for this article, as it leads to a better understanding of atomic structure compared to previous models. The unique feature of having a nucleus composed of protons and neutrons brought clarity to atomic mass calculations. Yet, the model does leave out the finer details of electron behavior, which paved the way for the development of quantum mechanics.
Bohr Model
The Bohr Model, proposed by Niels Bohr in 1913, presents a more advanced view of atomic structure focusing on electron orbits. Bohr suggested that electrons travel in fixed orbits around the nucleus and that they can jump between these levels. A key characteristic of the Bohr Model is its incorporation of quantized energy levels for electrons, adding a significant layer of sophistication to previous models.
This model is beneficial for this article as it aligns well with observed spectral lines of hydrogen, demonstrating its strong predictive power in explaining atomic emission and absorption spectra. The unique feature is that it successfully predicts the energy levels of electrons in hydrogen and allows for calculation of these levels. However, its limitations come to light when applied to more complex atoms, where it fails to provide accurate predictions due to its simplistic picture of electron movement.
Chemical Bonding Models
Chemical bonding models explore how atoms combine to form molecules, tackling concepts such as ionic, covalent, and metallic bonds. These models are essential for understanding molecular structure and properties. By examining these models, researchers can predict chemical behavior and reactions, which are foundational in fields like organic chemistry and materials science.
Thermodynamic Models
Thermodynamic models deal with energy changes during chemical reactions. They provide insights into spontaneity and equilibria within chemical systems. Understanding these models enables chemists to design reactions that optimize energy efficiency. These models are integral to understanding not just chemistry but also physical processes influencing various scientific disciplines.
Major Models in Physics
The study of physics relies heavily on various models that provide frameworks for understanding the complex behaviors and interactions in the physical universe. These models are not merely theoretical constructs; they bridge the abstract and the empirical, allowing researchers and students to conceptualize and predict physical phenomena effectively. They serve essential roles in education, innovation, and practical applications across many fields, such as engineering and environmental science.
Classical Mechanics Models
Newtonian Mechanics
Newtonian Mechanics is foundational in physics, focusing on the motion of objects under the influence of forces. Its primary contribution is in establishing the laws of motion and universal gravitation, which describe how objects move and interact. The key characteristic of this model lies in its simplicity and intuitive nature, making it a popular choice in educational settings as it lays the groundwork for more advanced concepts.
One unique feature of Newtonian Mechanics is its reliance on observable parameters like mass, distance, and time. Models derived from it can predict the motion of everyday objects with a high degree of accuracy, which is advantageous for practical applications, such as in vehicular motion and engineering designs. However, Newtonian Mechanics has limitations when dealing with phenomena at high velocities or in strong gravitational fields, where relativistic effects become significant.
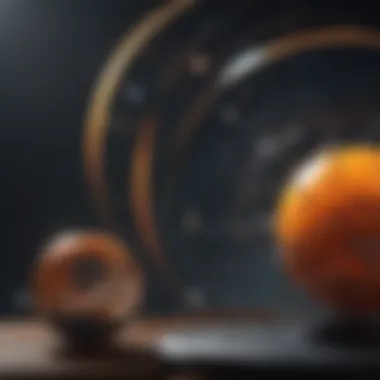
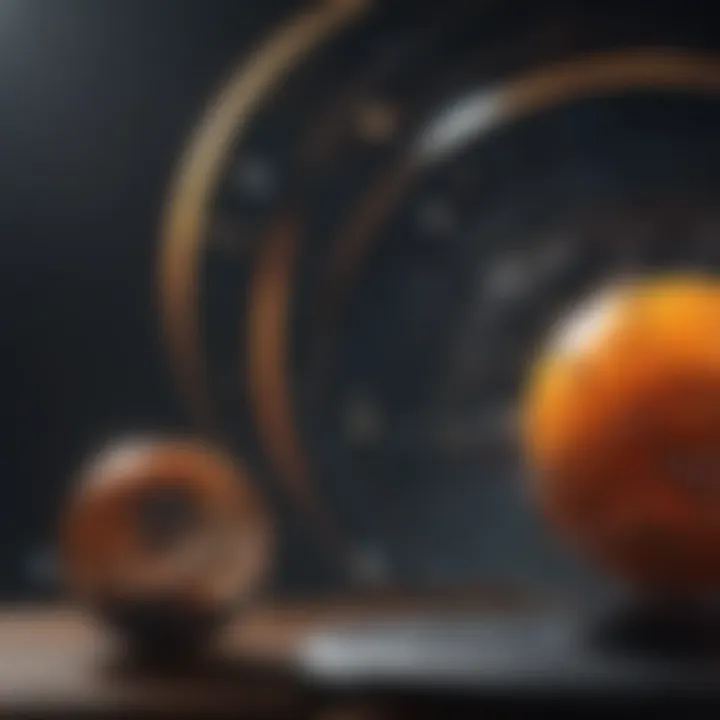
Lagrangian Mechanics
Lagrangian Mechanics is an advanced framework that reformulates the laws of motion established by Newton. It emphasizes an energy-based approach, focusing on the kinetic and potential energies involved in dynamic systems. This model's key characteristic is its use of the Lagrangian function, which is the difference between kinetic and potential energy. This makes it useful for solving complex mechanical problems where multiple forces are in play.
Lagrangian Mechanics offers a unique advantage in dealing with systems with constraints and conservation laws. It allows physicists to derive equations of motion without directly considering the forces acting on a system. However, the abstraction level is higher, requiring a solid understanding of calculus and variational principles, which can be a barrier for learners unfamiliar with sophisticated mathematical concepts.
Quantum Mechanics Models
Wave-Particle Duality
Wave-Particle Duality is a fundamental concept in quantum mechanics, suggesting that every particle or quantum entity can display both wave-like and particle-like properties. This notion has significant implications for our understanding of atomic and subatomic behaviors. The key characteristic of Wave-Particle Duality is its challenge to classical physics; it transforms how interactions at the quantum level are perceived.
A unique feature of this model is its implication that particles, such as electrons, can behave as waves, leading to phenomena like interference and diffraction. It is highly beneficial in explaining the behavior of particles in fields such as quantum optics and solid-state physics. However, this duality creates complexities when interpreting experimental results, as it defies classical intuitions about matter and energy.
Quantum Field Theory
Quantum Field Theory extends the principles of quantum mechanics by treating particles as excited states of underlying fields. It provides a unifying framework for understanding electromagnetic, weak, and strong forces. The model's key characteristic is its mathematical rigor, allowing predictions of particle interactions that align closely with experimental observations.
A significant advantage of Quantum Field Theory is its capacity to incorporate special relativity, thus addressing high-energy conditions and providing a robust foundation for particle physics. However, the complexity of its mathematics can be daunting, often requiring advanced studies in both quantum mechanics and field theory for full comprehension.
Relativity Models
Special Relativity
Special Relativity, proposed by Albert Einstein, redefined concepts of space and time. It introduces the idea that the laws of physics are the same for all observers, regardless of their relative motion. A key aspect of this model is its postulation that the speed of light is constant in a vacuum, leading to profound implications for our understanding of time and simultaneity.
This model is instrumental in explaining phenomena such as time dilation and length contraction. Its unique feature is that it unifies space and time into a single four-dimensional continuum known as spacetime. While immensely powerful, Special Relativity can present challenges in intuitively grasping non-classical concepts, making it less immediate for those encountering these ideas for the first time.
General Relativity
General Relativity further expands on Special Relativity, incorporating the effects of gravity into the framework. It describes gravity not as a force but as the curvature of spacetime caused by mass. This model's key characteristic is its geometric interpretation of gravity, allowing physicists to understand complex gravitational interactions within celestial bodies.
The unique feature of General Relativity is its ability to predict phenomena such as the bending of light around massive objects and the expansion of the universe. Though it's remarkably effective at explaining gravitational effects, the mathematical complexity associated with it can be a hurdle for learners, requiring familiarity with tensor calculus and differential geometry.
Major Models in Earth Sciences
The field of Earth Sciences encompasses a wide range of models that help in understanding the physical processes of our planet. These models play an essential role in interpreting geological, atmospheric, and hydrological phenomena. By providing frameworks that can predict natural events and human impacts, they offer systematic approaches to complex environmental questions. The models can be categorized into three primary types: the Plate Tectonics Model, Climate Models, and the Geological Time Scale Model. Each model offers unique insights, which helps in building a comprehensive understanding of Earth systems.
Plate Tectonics Model
The Plate Tectonics Model revolutionized our understanding of the Earth's structure and dynamics. It explains how the Earth's crust is divided into several plates that float on the semi-fluid asthenosphere beneath them. This model accounts for the movement of these plates and the resultant geological phenomena such as earthquakes, volcanoes, and mountain building.
One key characteristic of the Plate Tectonics Model is its emphasis on continental drift. This concept supports the idea that continents were once part of a single landmass and have since drifted apart. This model is crucial for predicting tectonic activity and understanding global geological processes, offering a unified theory for Earth's internal workings. However, it is not without limitations; for instance, it does not detail the forces driving plate movements in full.
Climate Models
Climate Models are vital for forecasting climate behavior and studying climactic variations across different timescales. They integrate principles from physics, meteorology, and oceanography, allowing scientists to simulate interactions within the Earth’s climate system.
Global Circulation Models
Global Circulation Models (GCMs) represent the most sophisticated tools for simulating climate systems on a global scale. They include complex mathematics to represent wind patterns, temperature distributions, and moisture levels across the planet. One notable advantage of GCMs is their capacity to integrate various factors, which makes them highly reliable in assessing future climate scenarios.
Despite their strengths, GCMs have limitations. They require extensive computational resources, and results can vary significantly based on input data quality. Furthermore, while they provide a broad view, they may not capture localized climatic effects accurately.
Regional Climate Models
Regional Climate Models (RCMs) focus on specific areas, providing fine-scale predictions that are often more informative for local stakeholders. These models excel in scenarios where detailed local data is necessary, such as agriculture or urban planning.
The primary characteristic of RCMs is their adaptability to include topography and local climatic patterns. As a result, they become especially useful in understanding regional climate variability. However, these models can struggle with data availability and may be less effective when scaling up to broader contexts, which can limit their applicability in global climate assessments.
Geological Time Scale Model
The Geological Time Scale Model serves as a framework for understanding Earth's history in chronological order. It divides Earth's 4.5 billion-year history into different eons, eras, periods, and epochs based on significant geological and biological events.
This model is crucial for correlating geological findings across various locations and helps scientists communicate complex historical data succinctly. By categorizing significant evolutionary milestones with geological events, it offers essential context for both natural history and earth sciences today.
The Geological Time Scale aids in understanding past climate conditions, which in return, can inform current environmental changes and guide future research. However, one must consider that the dating methods used to construct this scale can have uncertainties, affecting interpretations of the Earth's history.
"Understanding the major models in Earth Sciences is crucial for addressing contemporary environmental challenges. These models facilitate informed decision-making in areas like climate change and natural resource management."
In summary, each model within Earth Sciences plays a vital role in untangling the complexities of our planet. Their contributions extend from understanding tectonic shifts to predicting climate change impacts. However, it’s essential to be aware of their limitations and the challenges they present in application and interpretation.
Interdisciplinary Approaches to Scientific Modeling
In the landscape of scientific research, interdisciplinary approaches to scientific modeling are becoming increasingly vital. They serve to connect various scientific fields and enable more comprehensive understandings of complex phenomena. Collaboration across disciplines allows researchers to integrate diverse methodologies, theories, and perspectives. This integration not only enriches the analysis but also fosters innovative solutions to pressing scientific challenges.
Interdisciplinary modeling tackles problems that are too intricate to be solved by a single discipline. By pooling knowledge from fields such as biology, chemistry, physics, and earth sciences, researchers gain broader insights, leading to a more holistic view of the research topic. This collaborative effort is particularly important in addressing global issues like climate change, health pandemics, and sustainable energy.
Integration of Models Across Disciplines
The integration of models across disciplines plays a critical role in scientific inquiry. It enables researchers to transpose principles from one field to another, enhancing knowledge transfer. For instance, a concept from physics may improve a biological model's accuracy, or a chemical reaction could be analyzed using techniques from environmental science. Such interdisciplinary innovations lead to refined models that better explain and predict complex systems.
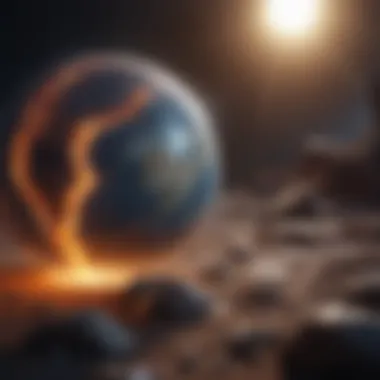
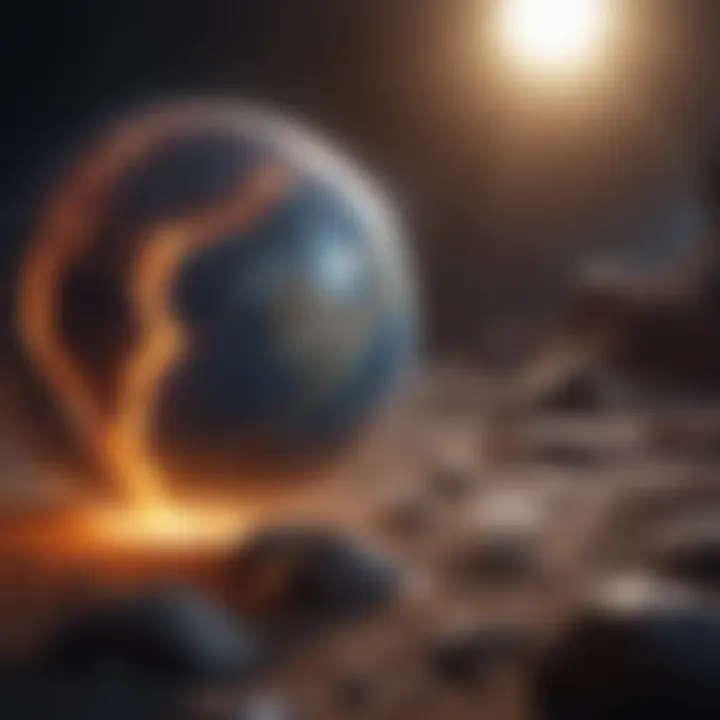
- Integration can help break down institutional silos that often hinder information sharing among scientific communities.
- Utilizing interdisciplinary models can result in more robust predictions, improving overall research effectiveness.
- Scientists can leverage computational tools developed in one field to analyze data in another, streamlining research workflows.
With interdisciplinary models, researchers have the opportunity to innovate and explore uncharted territory, fostering advancements that benefit society as a whole.
Application of Computational Models
The application of computational models has surged in recent years, driven by advancements in technology and data analysis. Computational modeling blends algorithms, simulations, and data analytics to create models that can predict outcomes across various scientific domains. These models are not just theoretical but are tested against real-world data, enhancing their reliability.
Moreover, computational models provide several benefits:
- Scalability: They can analyze large datasets faster than traditional methods, enabling quicker insights.
- Flexibility: Researchers can easily adjust variables to see how changes might affect outcomes, offering a dynamic platform for experimentation.
- Visualization: Complex data can be represented graphically, which aids in understanding and communicating findings effectively.
The use of computational models fosters collaboration and allows scientists to focus on creativity and critical thinking. As the models evolve, they bring new methodologies and insights that can lead to transformative discoveries in various fields.
In summary, interdisciplinary approaches to scientific modeling not only enhance collaboration but also drive progress in research through integrating models and leveraging computational powers. This interconnectedness among disciplines is essential for solving contemporary scientific problems.
Limitations and Challenges of Scientific Models
In scientific research, models serve as essential tools to simplify complex systems and phenomena. However, understanding the limitations and challenges of these models is equally important. Scientific models can enhance comprehension but can also mislead if not carefully utilized. Recognizing these limitations helps scientists and researchers to make more informed decisions in their work. Moreover, it aids in refining existing models and developing new ones that can better represent reality.
One significant challenge is the reductionist approach that many models take. This perspective breaks down complex systems into simpler components. While this can make the analysis more manageable, it often overlooks interactions among these components. Real-world systems are rarely linear and simple. Ignoring the nuances may lead to oversimplifications, causing models to fail in accurately depicting the phenomena they are intended to explain. Ultimately, such oversights can impede scientific understanding and progress.
Another critical element to consider is model accuracy and reliability. Even models that are well-constructed can yield different results based on the assumptions and parameters chosen. Variations in these inputs can lead to significant discrepancies in outcomes. Consequently, it is crucial for researchers to rigorously test and validate their models against empirical data. This process ensures that the models can reliably predict or interpret phenomena they are designed to explore.
In summary, while scientific models are indispensable in research, one must remain aware of their limitations and challenges. These factors can greatly influence the applicability and dependability of the models used in scientific inquiry.
Reductionism and Complexity
Reductionism is the practice of analyzing a system by breaking it down into its individual components. This approach has its benefits, such as facilitating focused studies on specific parts of a system. However, it can also be problematic. For instance, a model that only examines biological processes in isolation may neglect critical interactions with environmental factors.
When studying phenomena like ecosystems or human behavior, reductionism can lead to partial understandings. These models might miss underlying connections or synergistic effects that emerge at higher levels of complexity. As a result, researchers often struggle to translate findings from simplified models into broader contexts. Keys to addressing this limitation include:
- Holistic Approaches: Incorporating a broader scope may help represent interconnected elements more accurately.
- Systems Thinking: Evaluating the relationships and interactions among components can provide a more comprehensive view.
Model Accuracy and Reliability
Model accuracy plays a crucial role in scientific research, as it determines how closely a model reflects actual observations. Researchers must recognize that several factors affect accuracy. Common issues include:
- Assumptions: Many models rely on simplifying assumptions that may not hold true in real-world situations.
- Parameter Selection: Choosing the wrong parameters can skew results and lead to incorrect conclusions.
For a model to be deemed reliable, it should undergo validation processes. This includes:
- Empirical Testing: Comparing model predictions against real-world data helps assess accuracy.
- Reproducibility: Other researchers should be able to replicate results under similar conditions to confirm findings.
In essence, understanding the limitations stemming from reductionism and the critical need for accuracy are essential to scientific modeling. Models need constant evaluation and scrutiny. This ensures their continued relevance and effectiveness in advancing not just our understanding, but the broader field of science itself.
The Future of Scientific Modeling
The future of scientific modeling presents a pivotal opportunity for enhancing research methodologies across multiple disciplines. As the pace of scientific discovery accelerates, there is an intrinsic need for more sophisticated models that can replicate complex systems with greater fidelity. This evolution demands not just improvements in the models themselves, but also a broader perspective on how these models integrate with emerging technologies and new data sources.
One significant aspect of this evolution hinges on the integration of big data into modeling practices. The ability to analyze vast amounts of data will enable researchers to refine existing models and develop new ones that can better predict outcomes. For example, in fields like climate science, the use of high-resolution data from satellites and ground sensors will allow for more accurate climate models, providing critical insights into future environmental scenarios.
Additionally, advancements in computational power and algorithms, particularly in artificial intelligence and machine learning, will reshape scientific modeling. These technologies facilitate the exploration of data in ways that were previously unmanageable. By recognizing patterns that are not immediately obvious, AI-enabled models can generate valuable predictive insights that could transform research in several fields.
Emerging Trends in Modeling
Emerging trends in scientific modeling are characterized by innovation and collaboration. One notable trend is the shift towards integrative models that encompass interdisciplinary data and insights. As researchers increasingly recognize that many real-world problems cross traditional disciplinary boundaries, integrative modeling allows for comprehensive analysis that includes variables from various fields.
- Interdisciplinary Collaboration: Partnerships are fostering shared expertise and resources, leading to richer models.
- Open Science Initiatives: The movement towards open data and open-source modeling tools accelerates accessibility and transparency in research.
- Real-time Modeling: Advancements in computational technologies allow for modeling to be updated in real-time, enhancing the responsiveness of research.
- Enhanced Visualization Tools: Improved visualization techniques help communicate complex data and model outputs to a broader audience, including policymakers and the public.
These trends are reshaping the landscape of scientific modeling, thus equipping researchers with the tools to tackle multifaceted challenges more effectively.
Potential for Innovation in Research
The potential for innovation in research catalyzed by advancements in scientific modeling is profound. As models become more accurate and accessible, they will not only enhance the understanding of existing phenomena but also spur entirely new lines of inquiry.
- Predictive Analytics: More advanced models enable enhanced predictive analytics, providing foresight in areas such as health, environment, and social systems.
- Automation of Research: Automation will streamline data collection, analysis, and model generation, thus refocusing human efforts on more strategic and creative aspects of research.
- Ethical Considerations: As models evolve, it becomes critical to consider the ethical implications of their use, especially in sensitive areas like genetic engineering or climate intervention strategies.
The ability to create and refine models continuously means that researchers can expect to see groundbreaking discoveries emerge from their work.
By leveraging these innovative capacities, the future of scientific modeling is poised to not only enhance existing research frameworks but also usher in transformative changes within the scientific community. Adaptations to modeling practices will undoubtedly affect how we understand, interpret, and engage with the world around us.
End
In the conclusion of this article, we emphasize the profound significance of understanding scientific models across various disciplines. Scientific models serve as essential tools for condensing complex information into frameworks that can be tested and analyzed. They form the foundation upon which scientific inquiry is built, guiding researchers and scholars in their exploration of the natural world.
Summary of Insights
Throughout this article, we have examined several key models in the realms of biology, chemistry, physics, and earth sciences. The discussion has revealed how these models contribute to a deeper understanding of intricate phenomena in our universe:
- Biological Models: Models such as cell theory and evolutionary frameworks provide insights into the dynamics of life.
- Chemical Models: Atomic structures reveal the fundamental components of matter and their interactions.
- Physical Models: The laws of classical and quantum mechanics explain force, motion, and the behavior of particles.
- Earth Science Models: These models enhance our grasp of geological processes and climate mechanisms, essential for environmental science.
The synthesis of insights across these domains lays the groundwork for future research and collaboration, enabling a more integrated approach to scientific study.
Call for Continued Research and Development
The exploration of scientific models is not a finite endeavor; it requires ongoing inquiry and adaptation. It is essential to:
- Advance Methodologies: As technology evolves, so too should our approaches to modeling. Tools such as simulation software and computational models are revolutionizing research practices.
- Encourage Interdisciplinary Collaboration: Breaking down silos between scientific fields can lead to innovative models that address complex questions more holistically.
- Focus on Real-World Applications: Models developed in the lab must find pathways to practical application. This ensures that theories translate into tangible benefits for society, such as advancements in health, technology, and environmental stewardship.