Advancements in Predicting Proteolytic Cleavage Sites
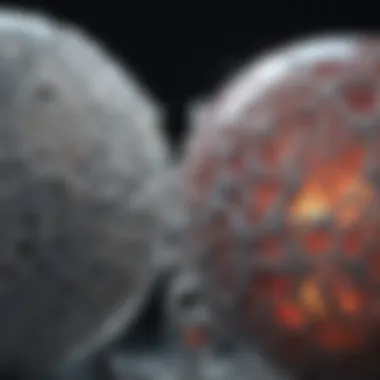
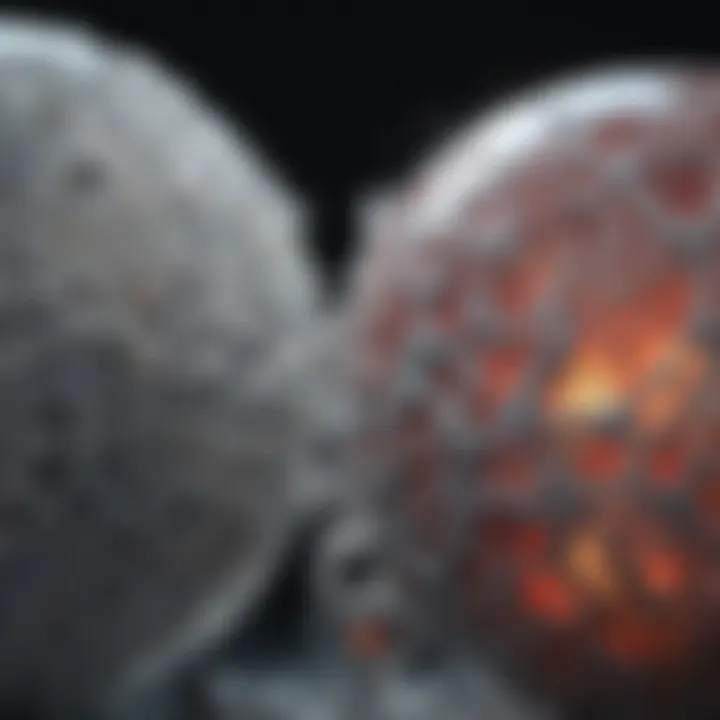
Intro
Proteolytic cleavage sites are fundamental components within proteins. These sites determine where enzymes such as proteases will cut a protein chain. Understanding how to predict these sites has broad implications in biochemistry, particularly in the development of therapeutic strategies. Accurate prediction of proteolytic cleavage can lead to significant insights into protein functionality and stability, as specific cleavages can activate or deactivate biological processes. Therefore, it becomes essential to systematically explore the methodologies and challenges associated with this subject.
This article will focus on the various computational models that can aid in predicting cleavage sites within proteins. We will delve into existing methodologies and their applications within drug development, highlighting relevant research patterns. Also, we will identify the continuing challenges that researchers face in this critical area of study. The objective is to synthesize information that not only enriches our understanding but also provides a pathway for future research.
Preface to Proteolytic Cleavage
Understanding proteolytic cleavage is essential for various fields of biological research. This article investigates the mechanisms, significance, and methodologies associated with predicting proteolytic cleavage sites within proteins. The process of proteolytic cleavage is a critical factor in cellular functions, impacting protein activity, stability, and degradation. By exploring the nuances of this subject, we gain insights into how these processes affect the broader landscape of molecular biology.
Definition of Proteolytic Cleavage
Proteolytic cleavage refers to the process through which proteins are hydrolyzed by enzymes known as proteases. This enzymatic activity results in the breaking of peptide bonds within a polypeptide chain. Importantly, this cleavage can lead to the activation or deactivation of biological molecules, resulting in significant physiological changes. In biochemical terms, the specificity with which these cleavages occur is determined by various factors, including the amino acid sequence surrounding the cleavage site and the active site of the protease involved.
Biological Importance
The role of proteolytic cleavage extends beyond mere protein activation. It plays a vital role in several biological processes such as digestion, cell signaling, and apoptosis. By regulating the activity of proteins, proteolytic cleavage ensures that biological pathways function correctly. Here are some key aspects of its biological importance:
- Regulation of Enzyme Activity: Many enzymes are synthesized as inactive precursors. Proteolytic cleavage converts these zymogens into their active forms.
- Cell Signaling: Cleavage events can produce signaling molecules that mediate key biological responses, influencing processes like inflammation and immune response.
- Protein Turnover: Cleavage facilitates the removal of misfolded or damaged proteins, thereby maintaining cellular health.
"Proteolytic cleavage is not only an essential process but also a fine-tuning mechanism for cellular regulation."
This multi-faceted biological importance underscores the necessity of accurate prediction of proteolytic cleavage sites. By mapping these sites, researchers can better understand the complex interactions within cells, paving the way for advancements in therapeutic development and biomedical research.
Understanding Cleavage Sites
Understanding cleavage sites is pivotal in the context of proteolytic cleavage. These sites are where enzymes, known as proteases, act on proteins to break them into smaller fragments. This process is fundamental for diverse biological processes such as digestion, cellular regulation, and apoptosis. Proteins are not static entities; their functionality often depends on the specific cleavage they undergo after synthesis. Characterizing these sites can reveal essential details about protein behavior and interactions.
Proteolytic cleavage can transform inactive precursors into active proteins. For instance, many hormones and enzymes are synthesized as inactive precursors and rely on cleavage for activation. Understanding the nature of these cleavage sites provides insights into how proteins operate within biological systems. This knowledge not only aids basic biochemistry but also informs clinical applications. For example, if we can predict where cleavage will occur, this could be crucial in drug design and development.
Furthermore, predicting cleavage sites allows scientists to optimize protein engineering. By knowing how modifications may influence cleavage, they can design proteins with desired functions. Consideration of how different factors, such as amino acid sequences and structural motifs, impact cleavage site prediction is critical.
This section lays the groundwork for exploring the role of proteases, as well as their types in the subsequent sections. By focusing on the characteristics of cleavage sites, researchers can make informed decisions regarding experimental approaches, ultimately enhancing prediction accuracy.
Role of Enzymes
Enzymes play a central role in proteolytic cleavage. Proteases, a specific group of enzymes, catalyze the breakdown of proteins. They are essential not just for digestion but also for cellular function and signaling pathways. Proteases can be classified into several categories based on their active sites and mechanisms of action. The regulation of these enzymes is equally crucial because improper cleavage can lead to diseases, including cancer and neurodegenerative disorders.
It is also important to differentiate between exopeptidases and endopeptidases. The former cleave at the ends of polypeptides, while the latter target internal peptide bonds. Their distinct functions highlight the complexity of proteolytic processes within cells. This complexity implicates enzymes significantly when considering prediction models, necessitating a thorough understanding of their behavior in relation to specific substrates.
Types of Proteases
Proteases are classified into several families based on their catalytic mechanisms. The most common types include serine proteases, cysteine proteases, metalloproteases, and aspartic proteases. Each group is defined by the amino acid at the active site responsible for catalysis.
- Serine Proteases: Utilize a serine residue to attack peptide bonds. They are vital in many biological processes, such as inflammation and blood coagulation.
- Cysteine Proteases: These enzymes use a cysteine residue for catalysis. They are often involved in protein degradation and processing cellular signaling.
- Metalloproteases: Require a metal ion, commonly zinc, for activity. They play roles in tissue remodeling and immune function.
- Aspartic Proteases: Utilize acidic residues (aspartate) to cleave peptide bonds. They are well-known for their function in digestive systems.
The diverse types of proteases emphasize the necessity for accurate prediction of cleavage sites. Different proteases have varying cleavage preferences, which adds a layer of complexity to prediction algorithms. Understanding these distinctions aids in refining computational models, leading us towards more accurate predictions in cleavage site identification.
Significance of Cleavage Site Prediction
The prediction of proteolytic cleavage sites is a vital area of study in molecular biology. Understanding where these cleavage events occur enables researchers to anticipate how proteins will behave within biological systems. This knowledge profoundly impacts various fields, including biochemistry and drug discovery, thus underscoring its significance. Predicting these sites accurately contributes to our overall understanding of protein functionality and stability, essential components of cellular processes.
Impact on Protein Function
The cleavage of proteins at specific sites has direct implications for their function. Proteins are often synthesized as precursors, and proteolytic cleavage activates or inactivates them. For instance, proenzymes or zymogens are inactive enzymes that require cleavage to reach their active form, highlighting the role of cleavage in regulating metabolic pathways. Misunderstanding these cleavage sites can lead to dysfunctional protein behavior, potentially resulting in various diseases.
Moreover, cleavage can alter protein interactions and localization within cells. Knowing where these events occur allows researchers to predict how proteins will interact with different cellular components. This understanding can be exploited for therapeutic strategies, such as designing inhibitors or activators targeting specific proteolytic enzymes.
Applications in Drug Design
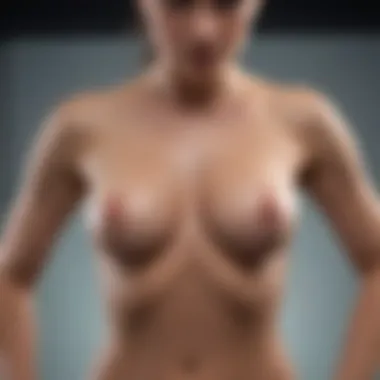
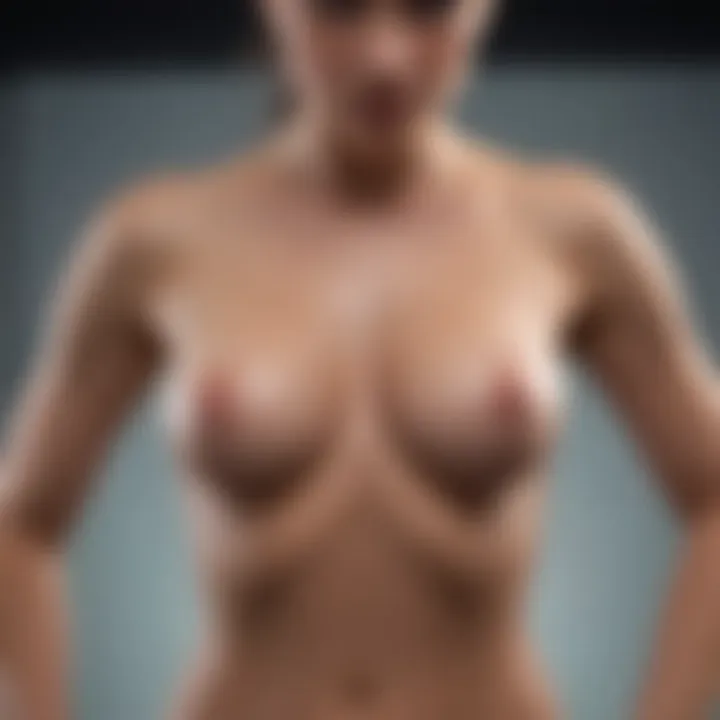
The relevance of cleavage site predictions in drug design cannot be understated. As biomedical research evolves, it becomes increasingly integral to develop drug candidates that achieve desired therapeutic outcomes by understanding and manipulating protein functions. Drugs targeting specific proteases can have profound effects by modulating protein activity, which can aid in treating various conditions, including cancer and cardiovascular diseases.
In addition, knowing the cleavage sites can help in designing biologics, such as monoclonal antibodies and peptide-based drugs. For example, targeting a particular proteolytic cleavage site can enhance the efficacy of a therapeutic protein, ensuring it is appropriately activated or directed to its site of action.
Understanding proteolytic cleavage sites is crucial in drug discovery. Proper predictions can lead to safer and more effective therapeutics.
Furthermore, computational models that incorporate cleavage site prediction can significantly streamline the drug development process. By integrating this data with other biological markers, researchers can better predict patient responses and personalize treatments. Overall, the significance of cleavage site prediction extends far beyond theoretical knowledge; it has practical implications in the advancement of medical science.
Computational Approaches to Prediction
Computational approaches play a crucial role in the prediction of proteolytic cleavage sites. Given the complexity of proteomic data and the need for accurate predictions, these methodologies provide both speed and precision. They allow researchers to analyze extensive datasets and identify potential cleavage sites that might not be apparent through experimental techniques alone. This section delves into two prominent methodologies: machine learning techniques and biophysical and statistical models, which have paved the way for advancements in this field.
Machine Learning Techniques
Machine learning has become an essential tool for predicting proteolytic cleavage sites due to its ability to learn from the data. By applying algorithms, these techniques can discern patterns within large sets of training data. The following points highlight the importance of machine learning in this context:
- Data-Driven Predictions: Machine learning models can adapt and improve as more data becomes available, offering a dynamic approach to predicting cleavage sites.
- Versatility: Algorithms such as Support Vector Machines and Neural Networks can be trained to recognize different types of cleavage patterns across diverse proteins.
- Integration of Features: Machine learning techniques can incorporate various features including sequence context, structural information, and evolutionary data.
Considerable work has been done using machine learning techniques to achieve better prediction accuracy. These advancements are significant because accurate predictions can inform our understanding of protein function and interactions. However, these models require careful tuning and validation to ensure reliability in various biological contexts.
Biophysical and Statistical Models
Biophysical and statistical models offer an alternative approach to predicting proteolytic cleavage sites. These models are often based on established biological principles and empirical observations. Here are some key aspects of these approaches:
- Theoretical Foundations: They utilize known biological mechanisms governing proteolytic activity, which can include enzyme-substrate interactions. Understanding these interactions helps to define potential cleavage sites.
- Statistical Analyses: By employing statistical methods, researchers can assess the likelihood of specific sites being cleaved based on historical data. Techniques like logistic regression and Bayesian analysis can be used.
- Simulations: Computational simulations can model enzymatic processes, providing valuable insights into how proteases interact with substrates over time.
Both biophysical and statistical models have their strengths in scenarios where detailed mechanistic information is available. They complement machine learning techniques, especially when integrated. This dual approach can lead to a more comprehensive understanding of cleavage site predictions and enhance overall accuracy.
The fusion of machine learning and biophysical models presents a robust avenue for improving our ability to predict where proteolytic cleavage occurs within proteins.
In summary, computational approaches are redefining how scientists predict proteolytic cleavage sites. As research continues to evolve, these methodologies will serve as the backbone for future advancements in understanding protein dynamics and function.
Data Sources for Prediction Models
The selection of appropriate data sources is vital for the development of accurate proteolytic cleavage site prediction models. High-quality data not only enhances the predictive capabilities but also bolsters the credibility of the findings. Several elements contribute to the importance of data sources in this domain:
- Quality of Data: Reliable and well-curated datasets provide a solid foundation for any computational model. Inaccuracies or noise in the data can lead to misleading results, affecting the overall efficacy of predictions.
- Relevance to Current Knowledge: The choice of data should reflect the latest discoveries and trends in proteolytic cleavage. Utilizing outdated or irrelevant information can hinder progress and obscure understanding of the subject.
- Diversity of Sources: Incorporating a wide range of data sources can improve model performance. Different datasets might provide insights into unique cleavage patterns and mechanisms, thus enriching the predictive framework.
For these reasons, concentrated efforts are put into establishing comprehensive data sources that researchers can access to enhance their models. The next sections will explore two essential types of data sources in detail.
Public Enzyme Databases
Public enzyme databases serve as a crucial resource for researchers focusing on proteolytic cleavage. These databases consolidate information on various enzymes, including their sequences, structures, and specificities. Notable examples include the BRENDA, MEROPS, and UniProt databases. Each of these repositories has its own strengths:
- BRENDA: Provides extensive information on enzyme functional data, catalysis, and kinetics. This database is helpful for understanding the biochemical activity of enzymes influencing cleavage sites.
- MEROPS: This comprehensive database focuses on peptidases and their inhibitors. It allows researchers to explore the classifications and specific activities of different proteases, which is essential for understanding cleavage mechanisms.
- UniProt: This resource offers a wealth of protein sequence and functional information. It is indispensable for researchers seeking to correlate sequence data with proteolytic behavior.
Accessing these databases aids in refining models by providing empirical evidence essential for predictions. Moreover, they allow for the testing of hypotheses concerning enzyme specificity and its implications in proteolytic cleavage.
Experimental Data Collection
Alongside public databases, experimental data collection remains a key component in refining prediction models. Experimental datasets typically emerge from laboratory studies designed to investigate specific proteolytic events. The importance of collecting experimental data is underscored by several factors:
- Validation of Computational Models: Experiments can confirm or reject predictions made by computational models. By comparing predicted cleavage sites against experimentally derived results, accuracy can be evaluated and adjusted.
- Insight into Novel Cleavage Sites: Through focused laboratory work, researchers often discover previously unknown cleavage sites. These findings can then be integrated back into predictive models, improving their predictive power.
- Resource for Training Machine Learning Models: Large volumes of experimental data can train machine learning algorithms, leading to improvements in their accuracy and robustness in predicting cleavage locations.
In summary, the use of experimental data complements information from public databases and enriches the field of proteolytic cleavage site prediction. This dual approach fosters more reliable models, leading to improved biological insights and applications in various research domains.
Challenges in Prediction Accuracy
Predicting proteolytic cleavage sites is not a straightforward task. Several factors contribute to the complexity of developing accurate prediction models. These challenges stem from the inherent variability found among proteins and the dynamic nature of their proteomes. Understanding these issues is essential as they directly affect prediction accuracy and the applicability of computational models in proteomics.
Variability Among Proteins
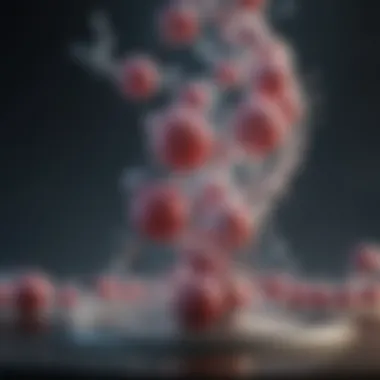
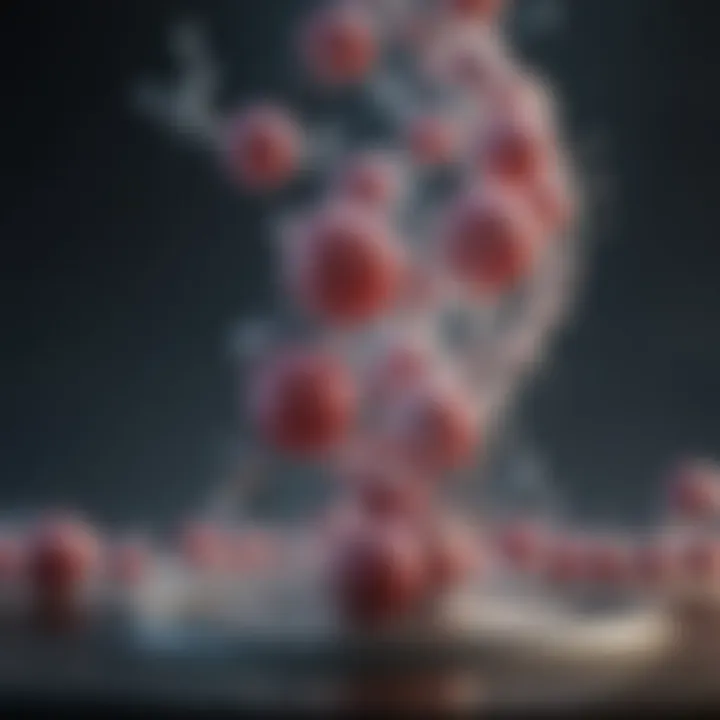
The variability among different proteins plays a significant role in creating challenges for cleavage site prediction. Each protein has unique sequences, structures, and functions, which can result in diverse cleavage patterns. For instance, a specific protease may cleave one substrate efficiently, while it may poorly interact with others. The discrepancies in cleavage specificity can arise due to factors such as the presence of amino acid motifs, secondary structure contexts, or even post-translational modifications.
Moreover, the diversity of Kleavage sites across different organisms adds another layer of complexity. For example, human proteins may exhibit distinct cleavage characteristics compared to their homologs found in bacteria or yeast. This means that a predictive model trained on a specific dataset may not be applicable universally. Thus, it is crucial to incorporate extensive datasets that encompass a variety of protein sequences from different sources when developing predictive tools.
Dynamic Nature of Proteomes
Proteomes are inherently dynamic. They can alter in response to various stimuli or during different physiological conditions. This variability can significantly affect the behavior of proteases, impacting how proteins are cleaved. For example, the activity of certain proteases can be modulated by factors like pH, temperature, or the presence of inhibitors or activators. Consequently, a cleavage site prediction model must consider not just the static sequence of a protein, but also the environmental context in which the protein exists.
Furthermore, post-translational modifications can drastically change cleavage outcomes. Modifications such as phosphorylation, ubiquitination, or glycosylation can influence the conformation of a protein, thereby affecting its interaction with proteases. As a result, the predictive accuracy may diminish when these factors are not integrated into the modeling process.
The dynamic nature of proteomes and variability among proteins pose significant hurdles for accurate prediction of cleavage sites.
To overcome these challenges, researchers are focusing on developing more sophisticated models that can integrate a wider array of biological data. This includes machine learning approaches that can capture complex interactions more effectively. Continuing to address these challenges can lead to improved prediction accuracy, ultimately enhancing our understanding of protease function and its biological implications.
Evaluation Metrics for Prediction Models
Evaluation metrics play a vital role in assessing the performance of prediction models in proteolytic cleavage site prediction. They provide a framework to measure how accurately a model can predict the cleavage sites from the protein sequences. A deep understanding of these metrics often facilitates the improvement of existing models and the development of new ones.
Sensitivity and Specificity
Sensitivity and specificity are two fundamental metrics used to evaluate the effectiveness of predictive models in identifying proteolytic cleavage sites. Sensitivity, also known as the true positive rate, measures the proportion of actual positive cases correctly identified by the model. In simple terms, it tells us how many of the true cleavage sites are found by the model. High sensitivity indicates that the model is proficient in capturing the majority of cleavage events.
On the other hand, specificity measures the proportion of actual negatives correctly identified. This means it indicates how many non-cleavage sites were correctly classified as such. A model with high specificity is essential to minimize false discoveries, which could lead to significant confusion during biological interpretation.
For a robust predictive model, a balance between sensitivity and specificity is needed. If a model is highly sensitive but lacks specificity, it may produce many false positive results. Conversely, a model with high specificity but low sensitivity fails to recognize true cleavage sites. Therefore, one should strive toward achieving optimal values for both metrics, enhancing the model's reliability.
Receiver Operating Characteristic (ROC) Curve
The Receiver Operating Characteristic (ROC) curve is a graphical representation that illustrates a model's diagnostic ability as its discrimination threshold varies. The ROC curve plots the true positive rate (sensitivity) against the false positive rate (1 - specificity) for various threshold settings.
An important aspect of the ROC curve is the area under the curve (AUC), which offers a single value representative of the overall performance of the model across all threshold levels. The AUC ranges from 0 to 1:
- An AUC of 0.5 suggests no better accuracy than random guessing.
- An AUC of 1 indicates perfect accuracy in distinguishing between cleavage and non-cleavage sites.
Using the ROC curve as part of model evaluation helps researchers visualize trade-offs between sensitivity and specificity. This visualization aids in selecting the right threshold for specific applications, where the consequences of false positives and false negatives may differ.
Emerging Technologies in Prediction
Emerging technologies are reshaping the landscape of proteolytic cleavage site prediction. As computational biology advances, new techniques have developed that enhance our understanding and ability to predict these critical sites. This section delves into two major emerging technologies: deep learning applications and the integration of multi-omics data, both of which offer significant benefits for the field.
Deep Learning Applications
Deep learning has become a cornerstone in modern computational biology. By mimicking the human brain’s neural network, deep learning algorithms can identify complex patterns in large datasets. In the context of proteolytic cleavage site prediction, these models can analyze sequences that traditional methods may struggle to interpret. They can capture subtle relationships between protein structure and function, leading to improved predictive accuracy.
- Increased Accuracy: Deep learning methods have shown to significantly outperform traditional machine learning techniques in various benchmarks. This improvement in accuracy is essential, as slight discrepancies in predictions can have notable biological implications.
- Large-Scale Data Handling: With the explosion of biological data, deep learning can manage large datasets, making it adaptable for high-throughput genomic studies. This capacity allows researchers to derive insights from vast biological datasets that previously might have been ignored.
- Real-Time Adaptation: Deep learning models can learn continuously. They can update their algorithms as new data becomes available, ensuring that predictions remain relevant and accurate.
Nevertheless, there are considerations with using deep learning, such as the need for extensive training data and computational resources. Careful attention is required in the training phase to avoid overfitting, where a model performs well on training data but poorly on new data.
Integration of Multi-Omics Data
The integration of multi-omics data, which encompasses genomics, proteomics, metabolomics, and transcriptomics, provides a holistic view of biological functions. This approach enhances the precision of proteolytic cleavage site prediction in several ways:
- Comprehensive Insights: By incorporating various omics data, researchers can observe a broader spectrum of biological interactions. This integrative method helps uncover the influence of other molecules on protein function and modification.
- Contextual Understanding: Understanding proteolytic processes in the context of cellular environments allows for more accurate predictions. Multi-omics enable researchers to factor in biological pathways and networks that determine how proteins behave in vivo.
- Enhanced Model Design: Multi-omics data can support the development of more sophisticated models. By accounting for additional layers of information, these models reflect the complexity of biological systems better than single-omics approaches.
The integration of multi-omics data represents a paradigm shift in how scientists approach the study of proteolytic cleavage.cite>
However, the integration process can be complex and requires continued development of methodologies that can effectively combine disparate data types. Researchers must navigate varying data scales, formats, and collection methods to fully harness the power of multi-omics.
Case Studies in Proteolytic Cleavage Predictions
Case studies serve as practical illustrations of theoretical frameworks in proteolytic cleavage site prediction. They provide real-world scenarios showing the implications of successful predictions as well as cautionary tales from failures. Understanding these elements deepens insights into methodologies, their operational realities, and the lessons learned from empirical evidence. The importance of this section lies in its ability to connect theoretical knowledge with practical application, thereby enhancing the reader's grasp of the topic.
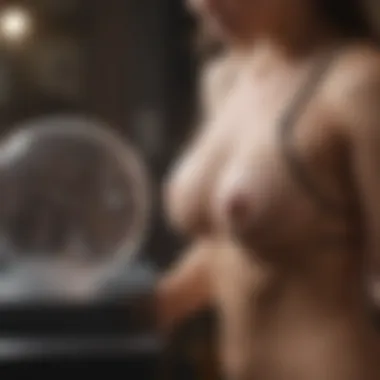
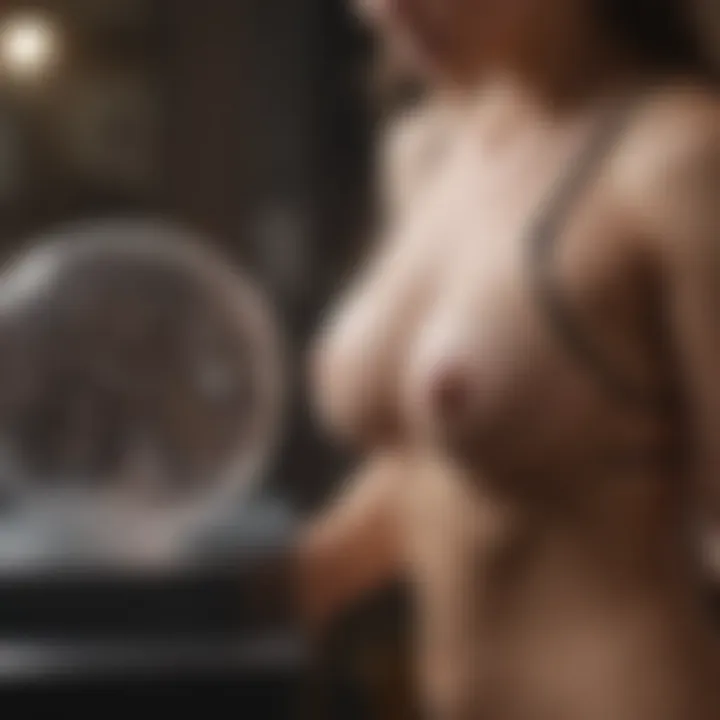
Predictive Success Stories
Predictive success stories in proteolytic cleavage predictions showcase instances where computational models accurately identified cleavage sites, leading to significant discoveries or advancements. For example, studies involving the identification of caspases and their cleavage sites have proven to be particularly fruitful. These predictions have contributed to improved understanding of apoptosis and the design of targeted therapies against cancer.
In another notable study, predictive methods were successfully used to discover cleavage sites in the HIV-1 protease. This knowledge was pivotal in the development of protease inhibitors, a class of antiretroviral drugs. By effectively predicting the sites, researchers could design drugs that specifically target and inhibit the activity of the protease, showcasing the real-world benefits of accurate predictions in therapeutic settings.
- Key Benefits of Success Stories:
- Demonstration of predictive models’ efficacy
- Contribution to drug design and development
- Enhanced understanding of protein dynamics and functions
The fusion of computational advances with biological insights has created avenues for ongoing research.
Lessons from Failed Predictions
Lessons from failed predictions in proteolytic cleavage site identification are equally crucial. They highlight the complexities involved in such biological predictions. For example, early computational models did not account for the structural diversity among proteases, which often led to unreliable predictions.
Another instance involved attempts to predict cleavage sites in neuropeptides. Initial models were unable to accurately identify where cleavages occurred due to the diverse environments and contexts in which these peptides function. These failures underscore the importance of integrating both machine learning approaches and biochemical knowledge.
- Considerations for Improvement:
- Enhancing model specificity to consider individual enzyme characteristics
- Incorporating more extensive datasets for training models
- Utilization of multi-omics data to improve accuracy
Future Directions in Research
As the study of proteolytic cleavage sites advances, several future directions merit attention. Understanding these avenues can refine methodologies and lead to breakthroughs in therapeutics and biochemistry. The convergence of various scientific disciplines provides a rich platform for enhanced research and application.
Potential for Novel Therapeutics
Potential for novel therapeutics is a significant outcome of improved clevage site prediction. Advances in this field could lead to identifying specific cleavage sites that, when targeted, may support the development of tailored drug therapies. These therapies could be more effective in treating specific diseases while minimizing off-target effects, a common downside of current treatment options. For instance, harnessing the mechanisms of protease inhibitors could open new pathways for managing conditions like cancer and viral infections.
Key benefits of exploring this potential include:
- Increased efficacy through precise targeting
- Reduced side effects by minimizing interactions with non-target proteins
- Improved patient outcomes with personalized medicine strategies
The integration of potential therapeutics with predictive models can guide researchers in understanding which proteins to target, ultimately leading to meaningful medical advances.
Enhancing Computational Techniques
Enhancing computational techniques remains critical for the future of proteolytic cleavage site prediction. Current methods, while innovative, still face challenges related to accuracy and generalizability across diverse protein families. Ongoing enhancements can address these issues, leading to more robust models and tools for researchers.
Some areas of focus for enhancement include:
- Incorporating Deep Learning: By leveraging deep learning frameworks, algorithms can be designed to recognize complex patterns within protein structures and sequences. This may enhance prediction accuracy.
- Improvements in Data Integration: Better integration of multi-omics data can enrich the models. This includes combining genomics, proteomics, and transcriptomics to reflect the dynamic behavior of proteolytic cleavage on a cellular level.
- Development of User-Friendly Tools: Creating accessible tools can encourage wider adoption in laboratories. Improved interfaces and software will help researchers without extensive computational backgrounds engage with predictive models.
The trajectory of computational techniques in this domain is promising. By enhancing them, the academic community can foster consistent and reliable predictions that inform both basic research and practical applications in health sciences.
"The importance of precision in proteolytic cleavage site prediction cannot be overstated; it not only provides insights into biological mechanisms but also fuels the development of more effective therapeutic strategies."
In summary, the future directions in proteolytic cleavage site prediction hold substantial promise. From novel therapeutics to advanced computational techniques, ongoing research will undoubtedly provide deeper insights into this intricate field.
Finale
In the realm of biochemistry, understanding proteolytic cleavage site prediction is paramount. This article has traversed various dimensions of this complex topic. The discussions provided a comprehensive understanding of the mechanisms, significance, and applications of predicting cleavage sites. The field stands at a crucial juncture where advancements in computational models and techniques can significantly enhance our understanding of protein function.
Summary of Key Insights
Throughout this article, key insights have emerged delineating the importance of accurate cleavage site prediction. Notable points include:
- The pivotal role of enzymes in defining cleavage patterns within proteins.
- Impacts of inaccurate predictions on drug design and therapeutic interventions.
- The integration of emerging technologies such as deep learning, which holds potential for improving prediction accuracy.
- Challenges posed by the dynamic nature of proteomes and diverse protein structures, necessitating ongoing research and development.
Understanding these aspects is critical for both researchers and practitioners in the field. As these insights are synthesized, they underline the necessity for robust methodologies in predicting cleavage sites, which could lead to scientifically and clinically significant breakthroughs.
Final Remarks on the Field
The field of proteolytic cleavage site prediction is evolving rapidly. Emerging technologies like deep learning and multi-omics integration promise new levels of accuracy and insight. As researchers continue to explore and refine computational approaches, the ability to predict cleavage sites will only improve. Meanwhile, challenges remain, especially concerning the variability inherent in protein structure and function.
Future research must aim to address these challenges while leveraging technological advancements. Continued exploration in this area has the potential to catalyze innovations in drug design and biotherapeutics, ultimately influencing how diseases are treated. The journey forward will be marked by both challenges and opportunities, underscoring the dynamic nature of science and its ever-expanding horizons.